Technical AI Governance
Governance of AI, much like its development and deployment, demands a deep technical understanding.
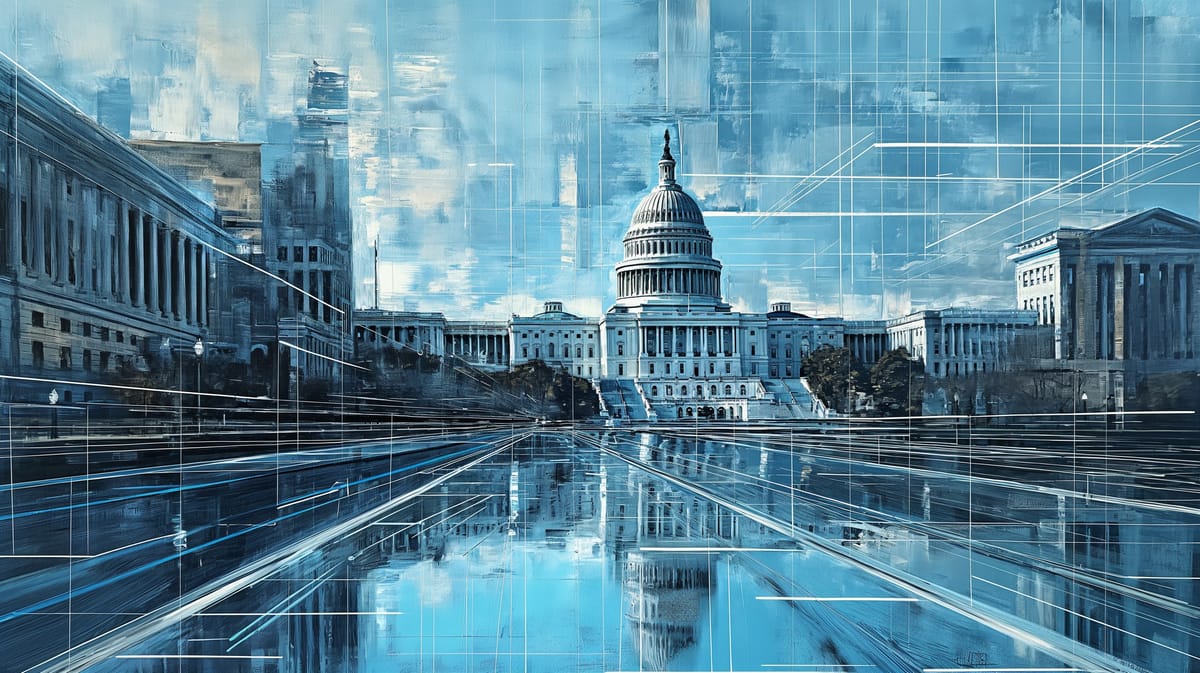
Governance of AI, much like its development and deployment, demands a deep technical understanding. This knowledge is essential for:
- understanding the current state and future direction of AI developments and
- designing effective AI governance policies by understanding technical details and/or enhancing them with technical mechanisms.
Both help to make more informed and more effective AI governance decisions. While these mechanisms do not directly solve the AI alignment problem[1], they are instrumental in managing the technology's impact.[2]
Recent U.S. government actions, including the October 7th, 2022, export controls[3] and the 2023 Executive Order on Safe, Secure, and Trustworthy AI[4], highlight the importance of technical expertise and analysis.[5] While these decisions were shaped by technical analysis, there remains a notable gap in the depth, breadth, and rigor of such analyses.[6] For instance, the discussion around compute training thresholds lacks depth[7], there is no accepted standard for measuring training compute[8], the analysis of chip specifications' impact on AI development and deployment is somewhat limited[9], and strategic considerations around hardware-enabled mechanisms[10] have not been explored.[11]
I primarily focus on understanding, leveraging, and governing compute - the computational power and infrastructure required for developing and deploying advanced AI systems.[12] In cutting-edge ML research, access to substantial computing resources and the skill to effectively utilize them have become almost synonymous with cutting-edge advancements.[13] Compute is a primary contributor to AI advancements, surpassing data and algorithmic improvements[14] — while also offering unique governance mechanisms.[15] As such, compute not only drives AI innovation but also presents unique opportunities for governance mechanisms.
The demand for expertise in this domain is evident. My engagements with government agencies, along with advising the leading AI companies, have demonstrated a clear market fit for this research and policy domain.
Technical AI Governance
At the core of my work is the technical analysis and understanding of advanced AI systems, which I refer to as Technical AI Governance.[16] This involves:
- (i) understanding the technical developments of AI at the frontier (e.g., What infrastructure does large-scale training require?) and
- (ii) improving our understanding of its trajectory, thereby enabling us to design better AI governance solutions (e.g., At which rate does compute investment efficiency increase?[17]).
- Additionally, my work encompasses (iii) designing technical mechanisms and standards[18] that supplement AI governance goals (e.g., proposing hardware-enabled mechanisms to supplement export controls).
These mechanisms are often not standalone solutions but rather tools that enhance the effectiveness of AI governance by providing assurances, building trust, or enabling new governance strategies.[19]
Technical AI Governance Research Definition
"Technical AI Governance research is the analysis of technical aspects of AI systems and AI R&D, as well as the design and analysis of technical mechanisms, to enable more effective and efficient AI governance tactics, policies, and strategies."
Compute Governance
Compute governance, a subset of Technical AI Governance, refers to the use of computational infrastructure as an instrument to enhance society's ability to govern AI. This involves increasing the visibility of AI systems to policymakers, allocating AI capabilities more effectively, and enhancing enforcement of norms and laws. It's important to note that compute governance extends beyond merely steering and limiting access to computational infrastructure; it includes using compute as a flexible instrument and measure for various governance purposes.[20]
Compute Governance Definition
"Compute governance refers to the use of computational infrastructure as an instrument to enhance society's capacity to govern AI, such as through increasing the visibility of AI to policymakers, allocating AI capabilities, and enhancing enforcement of norms and laws."
Compute governance is not self-sufficient — it needs to be embedded and leveraged in the wider AI governance context. This necessitates collaboration with others, where I contribute technical insights and tools (which may extend beyond compute) to support a wide range of governance efforts. While compute is currently a priority[21], I remain open to expanding my scope, utilizing any technical insights or tools that prove most effective.[22]
See, e.g., “The alignment problem from a deep learning perspective” (Ngo et al., 2023) ↩︎
See AI Governance Needs Technical Work, 2022 for more. ↩︎
For the original text, see Federal Register, 2022. For an analysis with relevant context, see Allen, 2022 ↩︎
Executive Order 14110 (Federal Register, 2023b). ↩︎
Many of the ongoing AI governance initiatives focus on national security. I think eventually we need to expand beyond just national security concerns. The diffusion of AI systems into our society and economy can and will have lasting impacts. We should also aspire to find solutions there. E.g., Value erosion, see Assadi, 2023 ↩︎
In contrast to AI governance policy decisions, where there may not be a single “best” governance framework, technical analysis often leads to more definitive conclusions. For example, the utility of a specific chip for a given AI architecture can be objectively assessed based on technical facts. ↩︎
E.g., some argue that compute thresholds could replace a capability assessment, whereas I see compute thresholds more as an initial filter to separate benign from potentially dangerous models. ↩︎
Regulations like the AI EO (Federal Register, 2023b) include language like “reporting requirements for any model that was trained using a quantity of computing power greater than 1026 integer or floating-point operations”. But how does one measure training compute? There are many computational processes during the lifecycle of an ML system, and ML systems often contain multiple ML models. For more discussion, see [redacted]. ↩︎
For instance, we have a limited understanding of how restricting export of certain chips and their specs affects the cost (in time or money) for training frontier systems. ↩︎
See “Considerations and Limitations for AI Hardware Mechanisms” (Heim, 2023j). ↩︎
This list of gaps is not comprehensive; these are just a few prominent examples. Additionally, these existing regulatory efforts only scratch the surface of ways that compute can be used to govern AI development and deployment. ↩︎
See “Computational Power and the Governance of AI” Sastry, Heim, Belfield, Anderjung, Brundage, Hazell, O'Keefe, Hadfield et al., 2024.. ↩︎
One way to see this is that, for cutting-edge models, computing is increasing at 4.2x/year, whereas dataset sizes are only increasing at 2.2x/year (for language models) and algorithmic improvements only decrease compute requirements by 3x/year (for image models). See Epoch, 2023. ↩︎
Relative to other key inputs to AI (data and algorithms), AI-relevant compute is a particularly effective point of intervention: it is detectable, excludable, quantifiable, and is produced via an extremely concentrated supply chain. We discuss this in “Computational Power and the Governance of AI” (Sastry, Heim, Belfield, Anderjung, Brundage, Hazell, O'Keefe, Hadfield et al., 2024.). ↩︎
This term also broadly encompasses the expertise required to contribute across diverse projects. It aligns with my past experiences, where the focus was on providing technical support and guidance. This involved diverse tasks such as ensuring accurate usage of technical terms and offering insights on technical possibilities and limitations in safety discussions, based upon a broad understanding of software and computer engineering. ↩︎
See Pilz et al., 2023. ↩︎
For more context and detail, see O’Keefe et al., 2022. ↩︎
My technical analysis does not encompass technical work aimed directly at aligning AI, i.e., modifying AI system behaviors. Nor do I fully develop new technical mechanisms. My role is more focused on conceptualizing and guiding these mechanisms rather than developing and implementing them, at least for now. ↩︎
E.g., compute governance also includes promoting responsible AI development by subsidizing compute access and using hardware-based verification for assurances. See Section 3 of “Transformative AI and Compute - Reading List” (Heim, 2023d). Further insights into the scope and motivation of our work can be gleaned from various resources like this presentation I gave at Google, or this transcript and recording of a previous talk for the scope and motivation of my previous and upcoming work. ↩︎
Also, the importance of compute hinges on various crucial considerations (Heim, 2023c). Its importance might be reduced over time and I am sensitive to the importance of problems we work on. ↩︎
Some topics beyond compute governance I could imagine working on include designing auditing infrastructure and information security. ↩︎