The Rise of DeepSeek: What the Headlines Miss
TLDR: timing is political but tech is real, compute constraints bite differently than you think, and the story is more complex than "export controls failed."
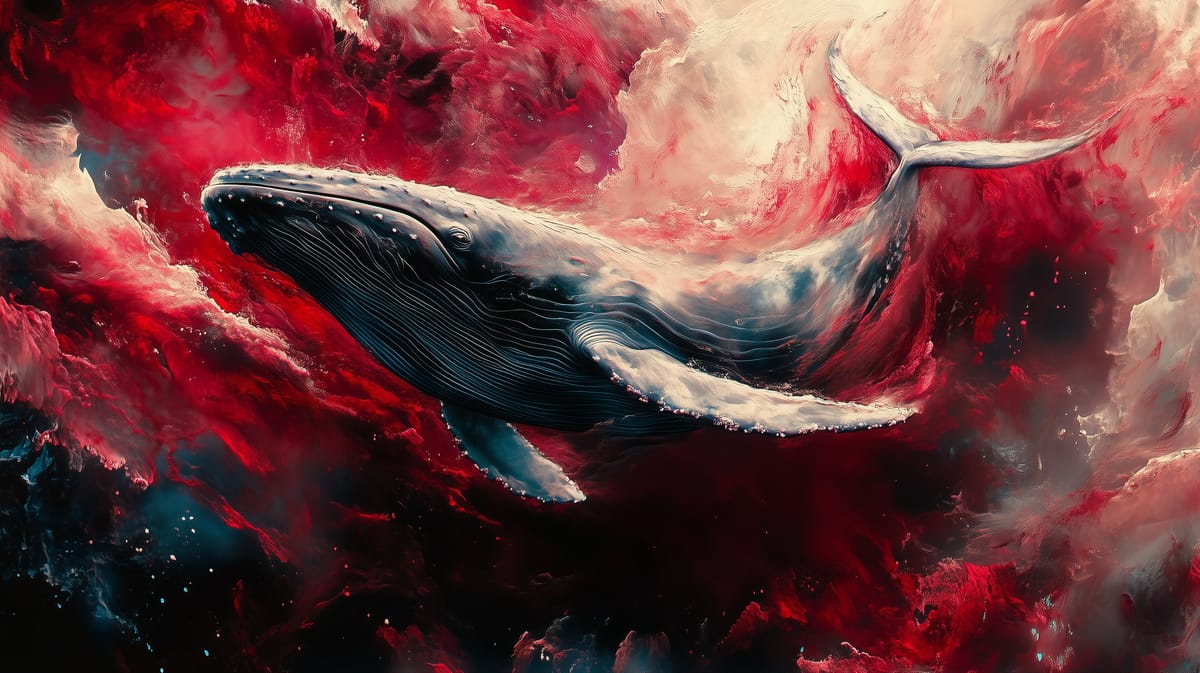
Lennart Heim and Sihao Huang
Recent coverage of DeepSeek's AI models has focused heavily on their impressive benchmark performance and efficiency gains. While these achievements deserve recognition and carry policy implications (more below), the story of compute access, export controls, and AI development is more complex than many reports suggest. Here are some key points that deserve more attention:
-
Real export restrictions on AI chips only started in October 2023, making claims about their ineffectiveness premature. DeepSeek trained on Nvidia H800s, chips designed specifically to circumvent the original October 2022 controls. For DeepSeek's workloads, these chips perform similarly to the H100s available in the US. The now available H20, Nvidia’s most recent AI chip which can be exported to China, is less performant for training (though it still offers significant deployment capabilities that should be addressed[1]).
-
Export controls on hardware operate with a time lag and haven't had time to bite yet.[2] China is still running pre-restriction data centers with tens of thousands of chips, while US companies are constructing data centers with hundreds of thousands. The real test comes when these data centers need upgrading or expansion—a process that will be easier for US firms but challenging for Chinese companies under US export controls. If next-generation models require 100,000 chips for training, export controls will significantly impact Chinese frontier model development. However, even without such scaling, the controls will affect China's AI ecosystem through reduced deployment capabilities, limited company growth, and constraints on synthetic training and self-play capabilities.
- The fact that DeepSeek V3 was trained on less compute is not surprising: machine learning algorithms have always gotten cheaper over time. But the same efficiency gains that allow smaller actors like DeepSeek to access a given capability (“access effect”) will probably also allow other companies to build more powerful systems on larger compute clusters (“performance effect”). We should be very glad that DeepSeek trained its V3 model with 2,000 H800 chips, not 200,000 B200 chips (Nvidia’s latest generation).
-
Their timing is strategic, but the technology is real. R1's release during President Trump’s inauguration last week is clearly intended to rattle the public’s confidence in the United States’ AI leadership during a pivotal moment in US policy, mirroring Huawei's product launch during former Secretary Raimondo's China visit.[3] This calculated PR timing shouldn't obscure two realities: DeepSeek's technical progress and the structural challenges they already and increasingly face from export controls.
-
It is harder for export controls to affect individual training runs, and easier to for them impact a whole ecosystem. Crucially, restrictions on the most advanced chips can effectively constrain large-scale AI deployment (i.e., allowing large numbers of users to access AI services) and capability advancement. AI companies typically spend 60-80% of their compute on deployment—even before the rise of compute-intensive reasoning models. Restricting compute access will increase the PRC's AI costs, limit widespread deployment, and constrain system capabilities. Importantly, deployment compute isn't just about serving users—it's crucial for generating synthetic training data and enabling capability feedback loops through model interactions, and building, scaling, and distilling better models.[4]
-
DeepSeek's efficiency gains may have come from previously having access to substantial compute. Counterintuitively, the path to using fewer chips (i.e., “efficiency”) may require starting with many more. DeepSeek operated Asia's first 10,000 A100 cluster, reportedly maintains a 50,000 H800 cluster, and has additional unlimited access to Chinese and foreign cloud providers (which is not export-controlled). This extensive compute access was likely crucial for developing their efficiency techniques through trial and error and for serving their models to customers.[5] While their R1 model demonstrates impressive efficiency, its development required significant compute for synthetic data generation, distillation, and experimentation.
-
The compute gap between US and China—further widened by export controls —remains DeepSeek’s primary constraint. DeepSeek’s leadership openly acknowledged a 4x compute disadvantage despite their efficiency gains. DeepSeek Founder Liang Wenfeng stated: "this means we need twice the computing power to achieve the same results. Additionally, there’s about a 2x gap in data efficiency, meaning we need 2x the training data and computing power to reach comparable outcomes. Combined, this requires 4x the computing power." He added: "We don’t have short-term fundraising plans. Our problem has never been funding; it’s the embargo on high-end chips."
One of their employees posted on X.com that “nothing can stop us on the path to AGI, except for computational resources.” This post was later deleted.
- Leading US companies keep the best of their capabilities private, meaning that public benchmarks paint an imperfect picture of AI progress. While some Chinese firms openly share their progress, companies like Anthropic, Google, and OpenAI maintain significant private capabilities. This makes direct comparisons based on public information incomplete. The attention on DeepSeek stems partly from their open approach—sharing model weights and methods in detail, unlike Western companies' increasingly closed stance. However, if openness necessarily translates to strategic advantage remains to be seen.[6]
So what?
DeepSeek's achievements are genuine and significant. Claims dismissing their progress as mere propaganda miss the mark.[7]
The reality of increasing compute efficiency means AI capabilities will inevitably diffuse. Controls alone aren't enough: they must be paired with actions to strengthen societal resilience and defense: creating institutions to identify, assess, and address AI risks and building robust defenses against potentially harmful AI applications from adversaries. However, we should also recognize that export controls already impact Chinese AI development and could have even stronger effects in the future.[8] While AI capabilities will likely diffuse regardless of controls—and it will always be difficult for export controls or other "capability interventions" to completely prevent proliferation—they remain important for maintaining our technological advantages. Controls buy valuable time, but need to be complemented with policies that ensure democracies stay in the lead and are resilient to adversaries.
ps. I (Lennart) also talked last Friday (Jan 24th) with Greg Allen from CSIS on his AI Policy Podcast about this.
The H20 chip, while restricted for training, remains uncontrolled and highly capable for frontier AI deployment, particularly for memory-intensive workloads like long context inference. This is significant given recent trends toward test-time compute, synthetic data generation, and reinforcement learning—all processes that are more memory-bound than compute-bound. Following the December 2024 restrictions on high-bandwidth memory exports, the H20's continued availability should be addressed, especially as deployment compute grows increasingly central to AI capabilities. ↩︎
Remember: this is all assuming export controls work perfectly—which they don't. We've seen plenty of loopholes in semiconductor controls and have credible reports of large-scale chip smuggling into China. While the Diffusion Framework should help plug some gaps, implementation remains a key challenge. ↩︎
The benchmark results of an r1 preview have already been public since November. ↩︎
E.g., see this recent Gwern comment that suggest that deployment compute plays a crucial role beyond just serving users. Models like OpenAI's o1 are used to generate high-quality training data for future models, creating a feedback loop where deployment capabilities directly enhance development capabilities and effectiveness. ↩︎
Recent usage spikes at other AI companies have led to service disruptions despite larger compute resources. DeepSeek's ability to handle similar surges remains untested and with limited compute they’ll face difficulties. (Sam Altman even claimed they are currently losing money on the ChatGPT Pro plan.) ↩︎
And we would love to see more discussions and analysis on this. ↩︎
Their reported training costs are not unprecedented given historical algorithmic efficiency trends. However, comparisons require careful context—DeepSeek only reports the final pre-training run costs, excluding crucial expenses like staff time, preliminary experiments, data acquisition, and infrastructure setup. See this post for a discussion at the top of how different cost accounting methods can lead to misleading comparisons. ↩︎
While models themselves may not be the strategic moat many assume, the compute impact on national security varies by use case. For use cases that require large-scale deployment (like mass surveillance), compute limitations could create significant barriers. For single-user applications, controls have less impact. The relationship between compute access and national security capabilities remains complex, even as model capabilities become more easily replicable. ↩︎