Compute and the Governance of AI - Talk
This talk explores the role of computational resources, or "compute", as a node for AI governance. Compute offers unique governance capacities for AI development and deployment that are otherwise challenging to realize.
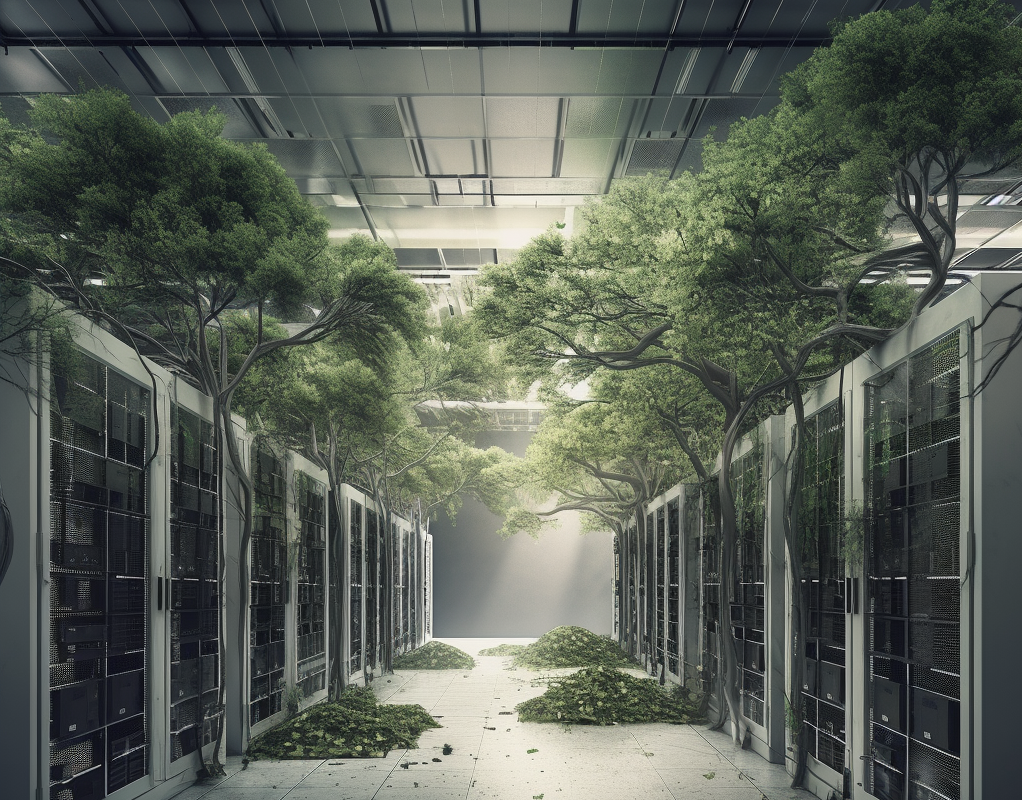
Find below a practice recording from the most recent version (Aug. 2023) of my talk on "Compute and the Governance of AI". Note that the transcript is AI-generated and probably contains some errors. You can find the slide deck here and added them below into the text.
Abstract
This talk explores the role of computational resources, or "compute", as a node for AI governance. Compute offers unique governance capacities for AI development and deployment that are otherwise challenging to realize. These include gaining insights into actors' current and future capabilities, guiding the distribution to foster development and deployment for beneficial use cases, and enabling effective enforcement against reckless or malicious activities. I will also examine how this approach fits into ongoing initiatives in the AI governance landscape. Additionally, I'll motivate some open technical questions that need to be addressed, such as using compute as an instrument to enable verifiable claims about AI development and use across different actors and states.
Video
Transcript
Hello, my name is Lennart Heim. I'm an AI Governance Researcher at the Center for the Governance of AI, where I'm mostly focusing on AI governance, particularly using computers and AI governance, which my talk will be about today.
Our work is focused on AI governance. I will define this later during the talk. And we also focus on more policy work, in particular in the UK, US, and EU, particularly in international efforts where we think these countries matter the most, and form part of decisions.
My background is actually in computer engineering so I'm coming from a technical background. I used to study at RWTH and ETH Zurich right here in Zurich and in my previous jobs, I mostly learned how computers work. Now I'm trying to bridge this gap between policy and governance and technical things and trying to find some niches there. So yeah without further ado let's just start, and jump right into the talk.
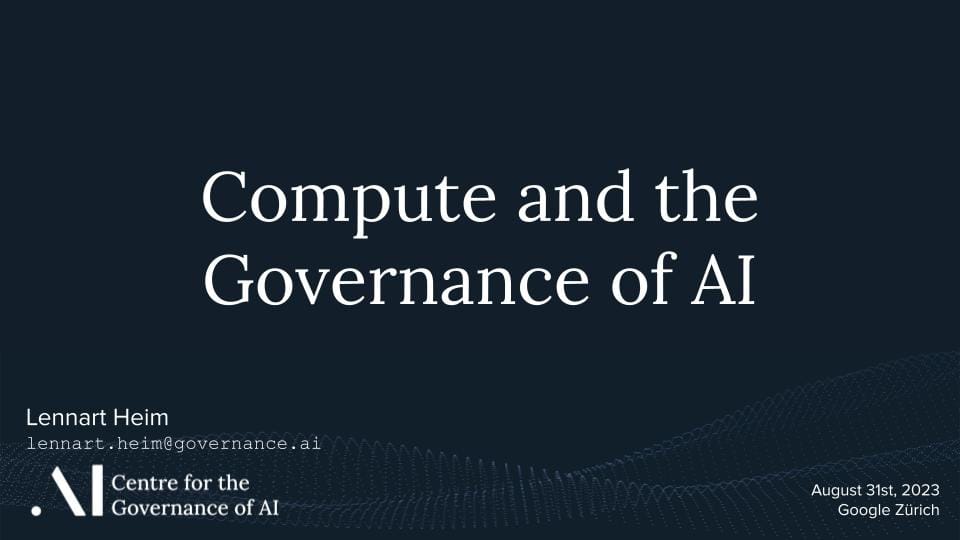
Okay, so the outline of my talk is roughly plan to talk for 30 to 35 minutes. Maybe I need to speed up a bit. Let's see just like where I stand. Maybe give me some gestures if you feel bored. I'm just repeating the same point over and over again. If I repeat a point a bunch of times I probably think it's important. I probably had some problems in the past that people didn't follow this point and I didn't take it as seriously as I want them to be. Then we can use the rest of this hour for a Q&A. In general, I’m also available via email or meetings at any time so don't worry if not.
I will start by basically introducing which kind of risks I see coming from advanced AI systems, and why I think we need governance institutions, policies, and laws. Then we'll particularly go to the promise of compute, why I think computers are particularly interesting to note of AI governance and important input to AI systems. Talking a bit about the theoretical and empirical aspects of this argument. I will then walk through, some like what I call AI governance capacities that compute enables which I think are unique and we do not get via other inputs and then just give some examples of compute governance already happening in the real world or other initiatives that I'm particularly excited about which I think would help with the bigger challenge of the AI governance problem. And then I will take a step back again and just look at like, well all of the things which as I said how does this fit into the large AI governance here, what are the things we can do today, do tomorrow and what are the ideas people have been advocating for.
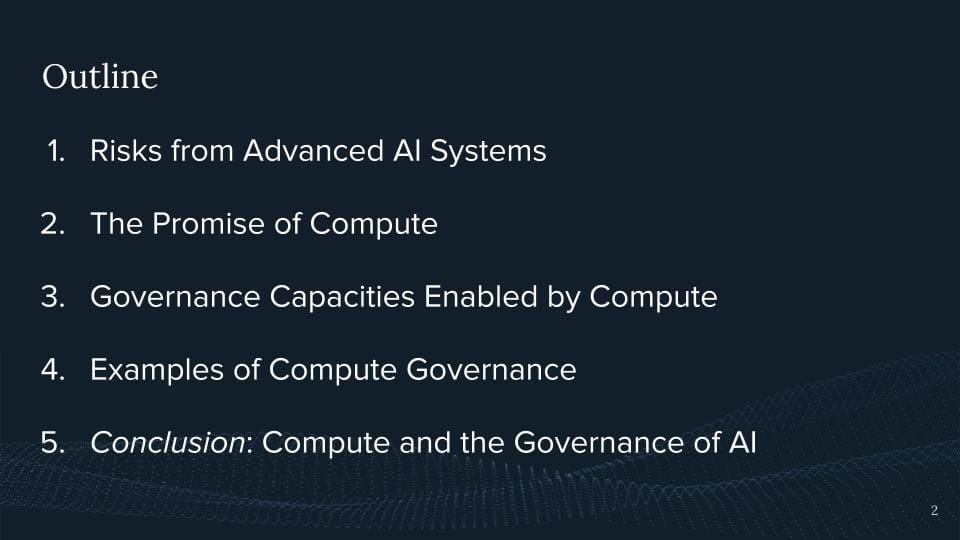
So let's get started with Risks from Advanced AI, outlining a bit of the problem statement.
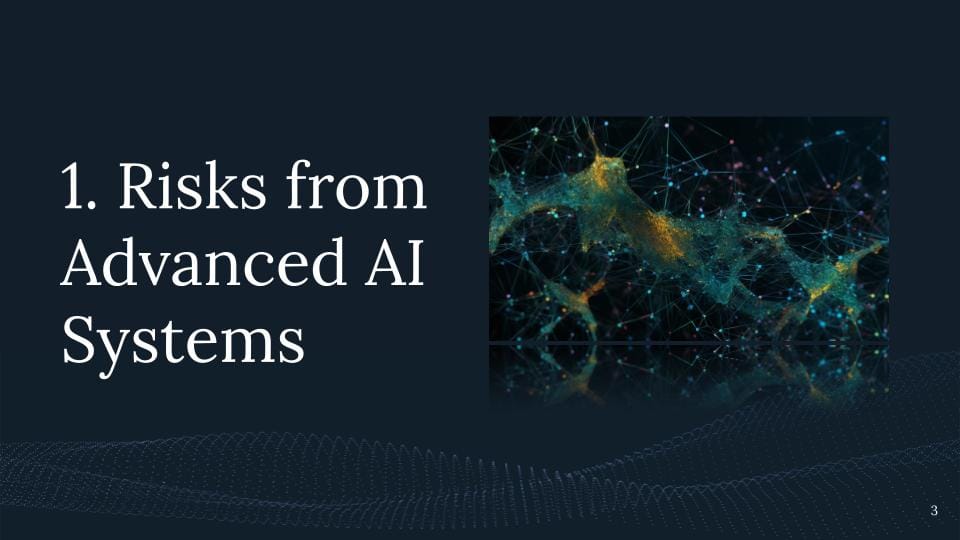
I think we're all somewhat aware of the rapid progress of AI systems, right? Of course, there's not a single chart that highlights it the best but I do like this one which is I chose to perform it across various benchmarks and then try to make it relative based on a human performance.
These AI capabilities are advancing rapidly. We talk about the last two, decades where we have just seen AI systems surpassing humans in some domains and just more about to come. And I think, yeah, we will track that many more will come.
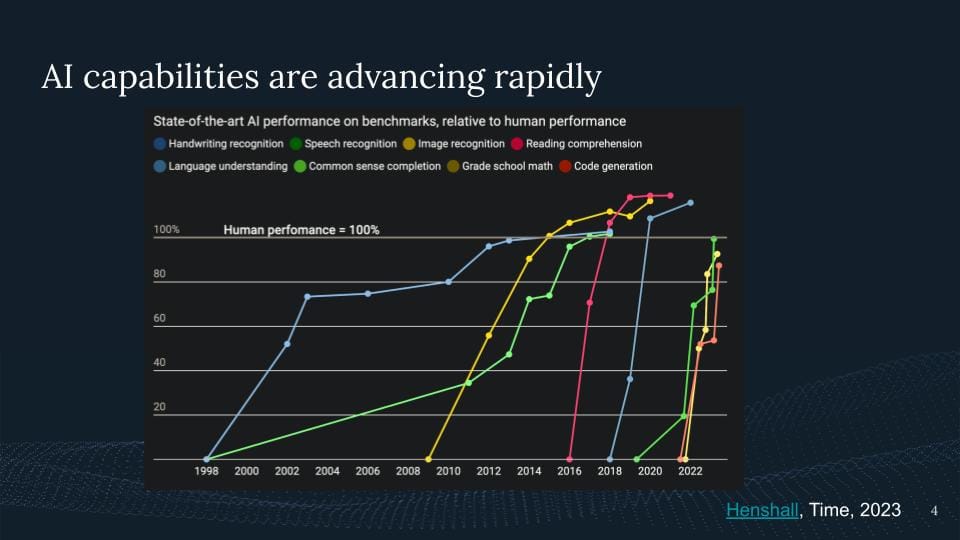
So why is this of concern? Well, if something is rapidly evolving, we should be aware of these risks. And I think what I wanted to do here is we can roughly think about three groups of risk for AI.
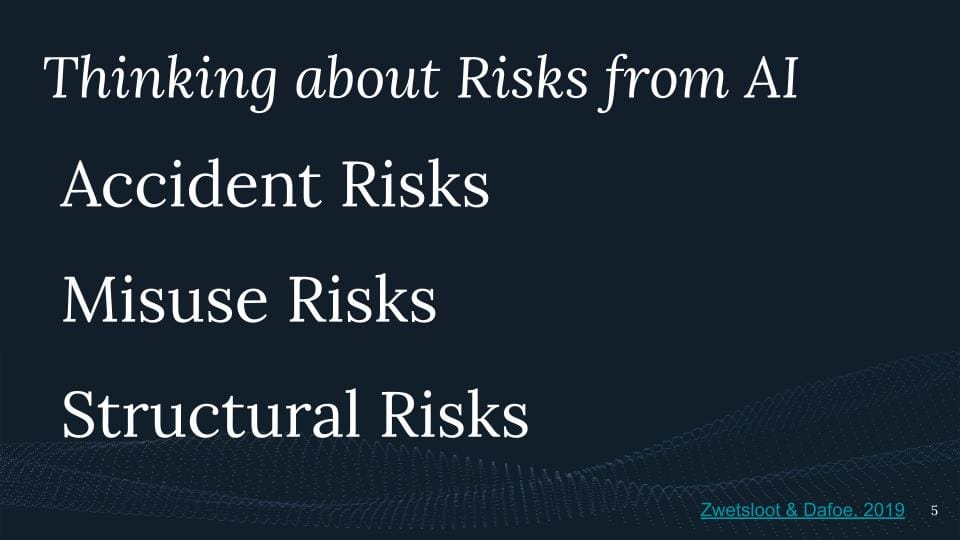
For a study, the Accident Risks.
It's like typical harm arising from AI systems behaving in unintended ways. A typical example would be a self-driving car crashing into something. Things that nobody intended to do, but they sometimes happen and there's harm could take place because of this.
Then there is second, there is Misuse Risks. This entails the possibility that people use systems in a way we didn't intend to use them. They're misusing the systems for whatever kind of bad purposes or malicious motivations they might be having.
And then third the Structural Risk, which I think is often the overlooked one and often the missed one, where we ask the question like how does technology shape the broad environment in ways that could be disruptive or harmful, more subtle changes but which eventually play out in the long run to play, be like really important. For example, asking the question, do AI capabilities produce dual-use capabilities? Does it lead to greater uncertainty or misunderstanding? Does it have new trade-offs regarding privacy and harm? Does it create conflict? Does it make wars better? What's the impact of nuclear warfare based on AI? What about competition? Is there a winner that takes all in AI? All of these questions are important. It poses a risk that AI systems pose eventually.
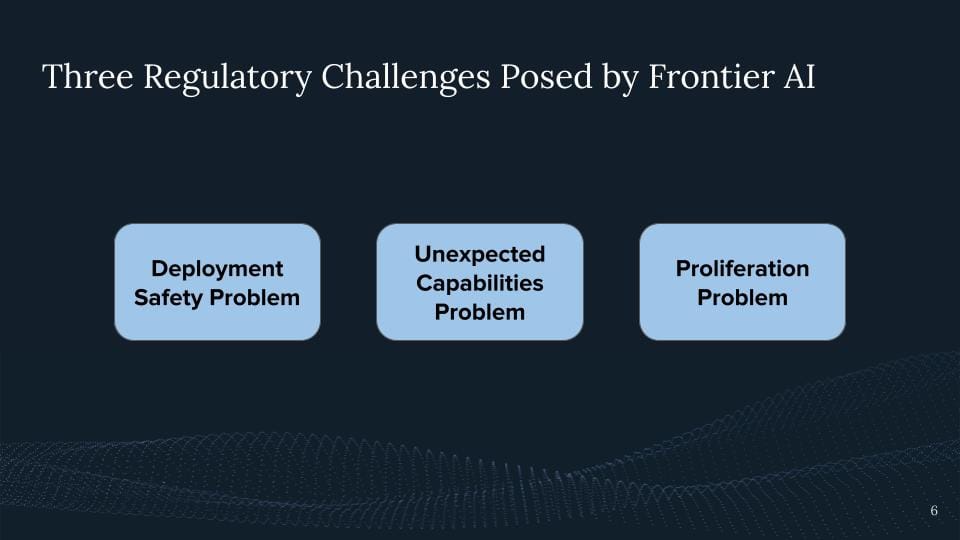
Another way to think about this is just like thinking about the regulatory challenge we face right now or what we'll probably be facing in the future, highlighted by some colleagues of mine in a recent paper.
So the three groups that highlight there is like first the deployment safety problem, where we highlight it's like kind of hard to say when we talk about large language models, which outputs are even harmful, right? And like given the jurisdictions which are, which other ones we want to like to prevent. But also even if you have misuse prevention in place, they might be wrong, right? We've seen it most recently with all the chat GPT with Bing, with all of these jailbreaks of these models.
And secondly, we have this unexpected capability problem. We have it, first of all, when we scale the systems, we have something like scaling laws where you can pretty nicely predict the log loss but this does not predict the capabilities downstream for a certain task, right, these will sometimes come unexpected and emerge suddenly and that's the thing which we're going to be on the lookout for, right. But also we have unexpected capabilities when we deploy the systems, right, when we make a system available to millions of users in the world, they find new ways of using the systems and find new unexpected capabilities. I think there's a bunch of applications we've all not had some foreseen which now are building on top of GPT-4 and our AI systems being out there.
And then lastly we have some proliferation problems. Some of these models are being outsourced so some everybody can use them. This might be okay now but the question is will this be okay going into the future? In particular, because it's a decision that is hard to reverse. And then also just like people reproducing capabilities. You just like you publish papers, you talk about these systems, other people are getting excited about this, other companies are getting excited about this, but also other nations say they might get excited about this. So they might just see the capabilities, and new algorithmic insights they might learn from, but in the worst case, these models can even potentially be stolen and be hacked. We could have a theft of this model, and then all of the upfront investments can be easily or cheaply used for potentially malicious actors.
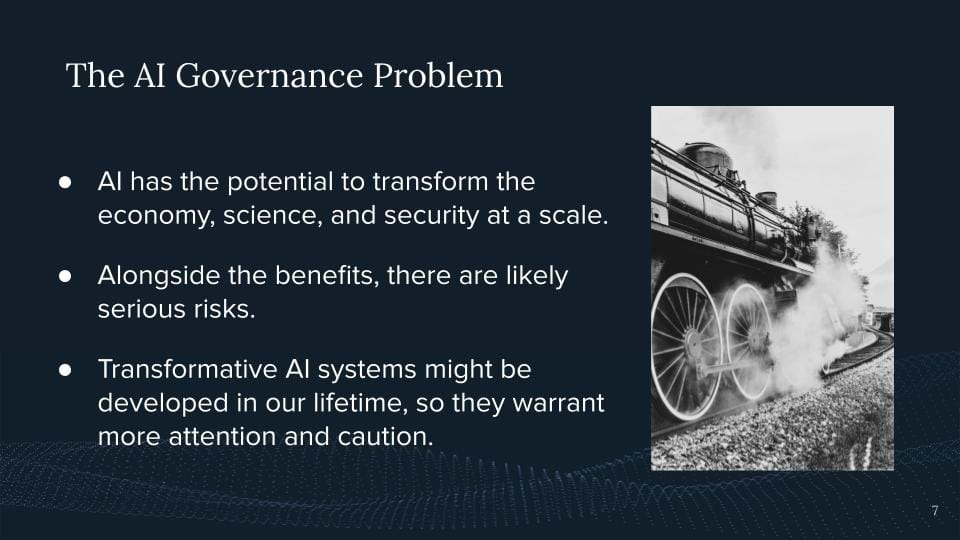
So to summarize, I think all of this combined, I'm claiming that AI has the potential to transform the economy, science, and security at a scale. There are probably huge benefits for society, but also some serious risks, like misaligned AI systems, concentration of power, and in general, just destabilization. And we've seen large increases in AI companies over the last decade. And so I think these transformant systems may develop within our lifetime. So risks like these seem worth giving significantly more attention.
I'm not saying all of us should not re-stir at what's working in this. All that I'm seeing is the research in AI governance and this problem has been getting way more attention over the last six months. But in the grand scheme of things, most people are still pushing the frontier of AI capabilities, and not that many people think about the risks and how we can manage, these kinds of systems as a society. So we don't know where this is gonna go, right?
The future is uncertain, but I think it would be naive to just, and wrong to ignore these types of risks. We should think carefully. And it's, in my opinion, kind of clear that we're currently not on track to solving these types of challenges.
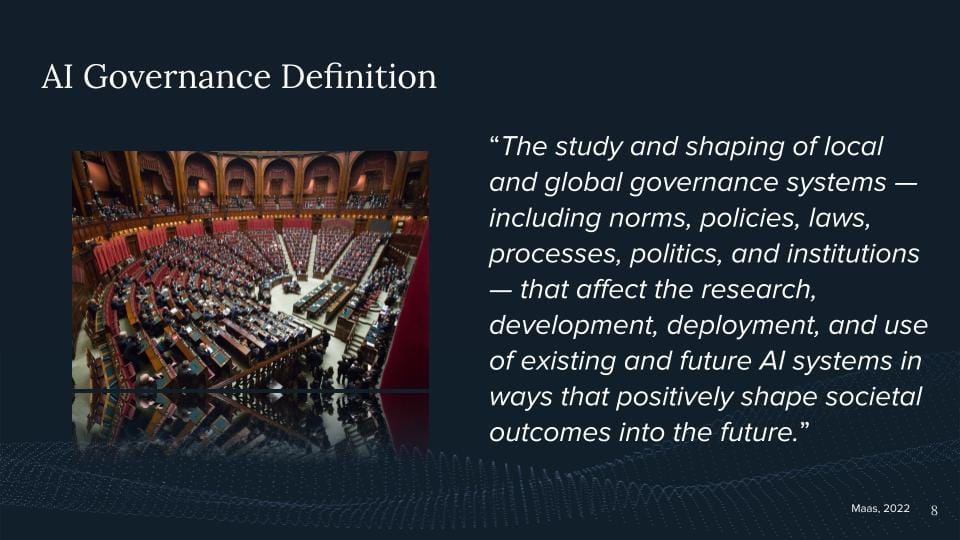
So, I will close the section with just a broad definition of AI governance to give you some kind of background on what I'm trying and what others are trying to achieve here. And that is an interdisciplinary field and I won't cover all of this in a talk. All that I will cover is like using one instrument which helps with all of these things.
So the definition here is the study and shaping of local and global governance systems, including norms, policies, laws, processes, politics, and institutions that affect the research, development, deployment, and use of existing and future AI systems in ways within eventually positively shaped the societal outcomes into the future.
So again highlighting that this is deep down an interdisciplinary problem, we need many many domains here and many many ways how we can know this. AI governance starts with you guys with the developers of these systems, within these companies which kind of risk management we have in place there but it also then goes further as to like our governance institutions in the world up to international institutions which need to eventually deal with these kinds of challenges, where we need to set up new policies and norms institutions to deal with this.
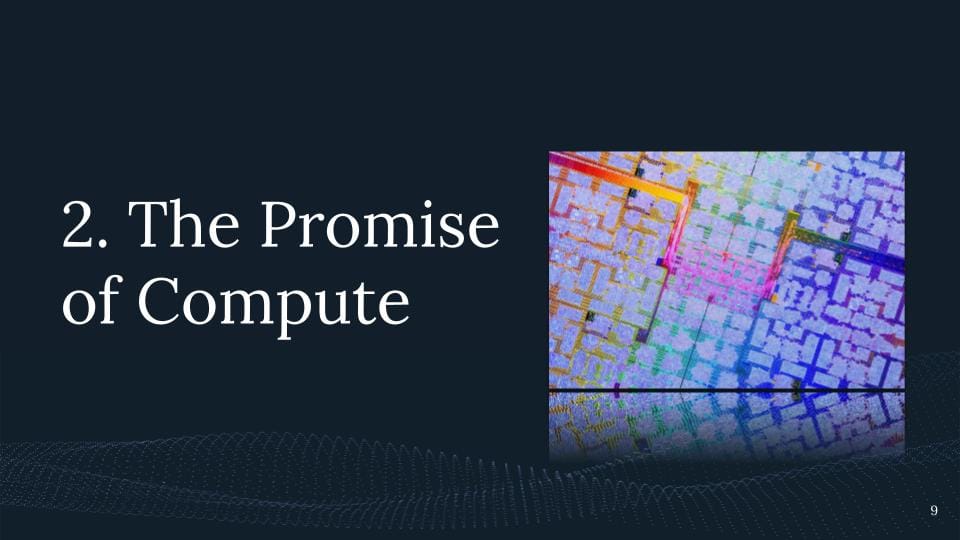
Okay, now I want to go to more content of my talk. I want to talk about the promise of compute, like using compute as a computing power in AI Governance.
So first I should probably define what I mean by compute. It's become, I think two years ago nobody used to compute and like this thing which everybody uses. I roughly mean computational power and computational infrastructure which powers our AI systems nowadays. So I'm talking about data centers full of hundreds to thousands of specialized AI chips which basically provide the power for training and deploying these models nowadays at scale.
I'm not talking about your smartphone even though it might have an AI co-processor on it. I'm mostly talking about the big supercomputers that enable these types of systems, these general-purpose systems that we see nowadays here. Of course, there are many limitations and all AI systems need a lot of compute. We'll go a little bit into this. With computing power, I mean, with computing power, the computational infrastructure required for this.
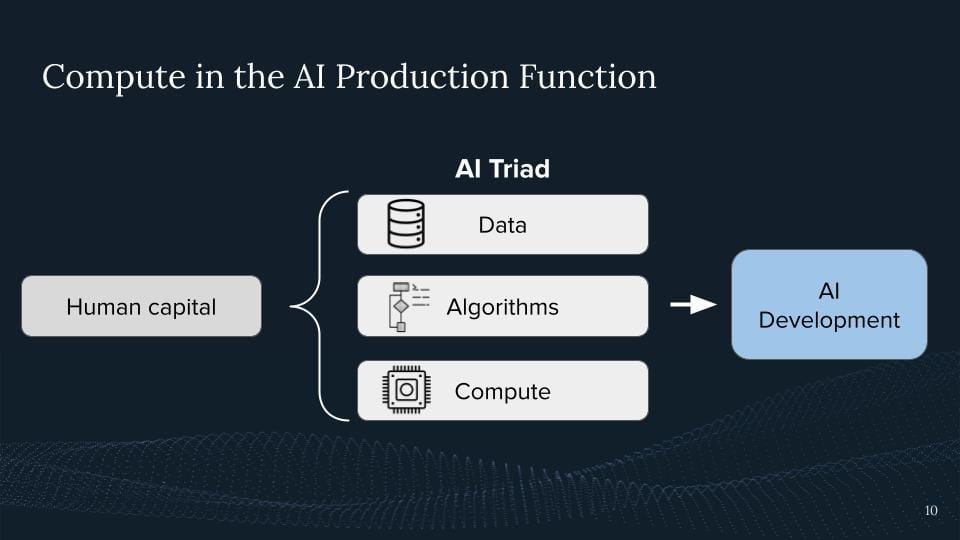
What is the role of compute in AI development?
What is the AI production function?
I roughly describe something where you have certain inputs, then you have this production function you get an AI model. And I think one way to describe is we start with human capital, right? That's where it all starts, that's us, who are developing these ideas. And I think one way to break this down is like, well, we start with data, right? We need like big datasets to obtain these modern systems. This is eventually collected, and and and created by humans. And this is part of this input AI system.
If you go further, we have the algorithms, right? From really the underlying nature of machine learning, neural networks, and deep learning, up to the transformer architecture up to somebody coming up with like a new loss function for creating the systems and making them a tiny bit better.
And then Lastly my claim is the other input well is the computational infrastructure which we then need to run these algorithms which are then being trained on this data and out we get is like OAI model or like the general purpose of AI development. So I think it's a good idea to think about this AI triad where we have data algorithms and compute and my argument is that compared to the errors computers are particularly interesting and bring certain governance nodes and tools which others do not bring to the table. Therefore, it's particularly useful for AI governance.
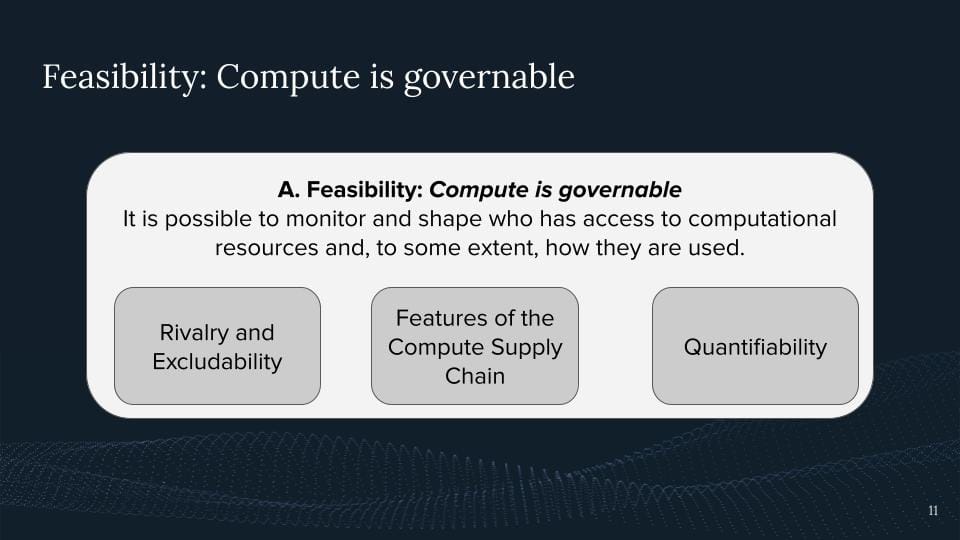
So I'm claiming that compute is governance. With this mean, I roughly mean something as well. It's possible to shape and monitor with access to computer resources and to some extent how they're being used.
Why do I think so? I think the first argument is rivalry and excludability.
Rivalry describes if I'm using something, if I'm eating a banana, you can't eat it anymore. That's not necessarily the case for data algorithms, right? Data algorithms just command C, and command V, we got it twice. My usage does not exclude your usage. Whereas for compute this is the case, right? If I'm running a model and I'm fully utilizing a GPU, nobody else can use it. It's only that many floating point operations per second in this GPU can provide, right? Right now at this moment, there's a limited amount of computing power in the world and it's being used in certain ways and it's not unlimited to that extent, right?
And the excludability describes where we have ways to expel people from using this compute. This can mean we just don't give them access to the chips, or in the case of cloud, computing we can just kick somebody off of the host if we want to. However, this is potentially way harder with data and algorithms to score a little bit worse on these kinds of dimensions.
The other one is the features of the supply chain. I'm going to go too much into the weeds because I got some slides on this later where I talk about these features. But creating compute is a complex product within multiple choke points across the supply chain which you can leverage for AI governance eventually.
My other argument is the quantifiability. I can count chips, two actors with one with 100 chips, the other one with 200 chips if these are the same chips. I claim the one with more chips will have more access to AI capabilities eventually. Not necessarily. I always can make a difference here, but all things being equal, more computing with more power in this case. We can similarly nicely count it based on the number of chips, but also based on the computational performance of the chips, based on how much memory, bandwidth, you name it, all of the specifications, right? This is eventually a product we have. This is way harder to do with data. We have some quality dimensions, we have some bias dimensions, many many dimensions, and many people are trying to quantify this better.
I claim that computers are a little bit easier. And if the algorithm gets even harder, right?
How can we measure one algorithm better than the other? Eventually, one way we often measure this is which algorithm with less compute gets better capabilities, right? So it goes down to how can you use compute and computing power more efficiently. My claim is, that these three properties may compute particularly governable.
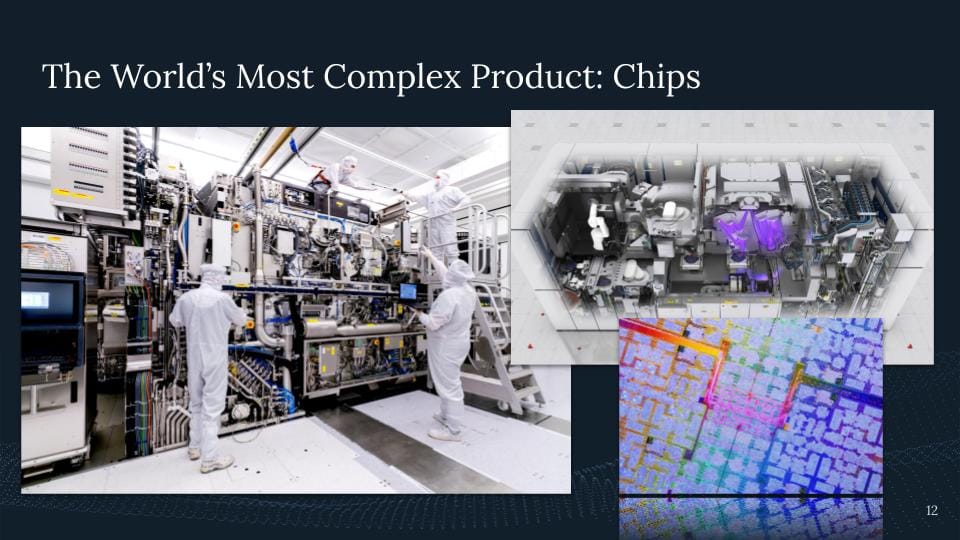
Let's dive a little bit deeper into the supply chain. So I've already hinted at it. I do think chips, AI chips are probably the most complex product in the world. What we see on this picture, here on the right-hand side, I think it's an NVIDIA A100 or P100, just a die shot of it. And then we see ASML building a so-called EUV machine, an extreme ultraviolet lithography machine, which is then producing these kinds of chips, where it's only one company in the world eventually producing these.
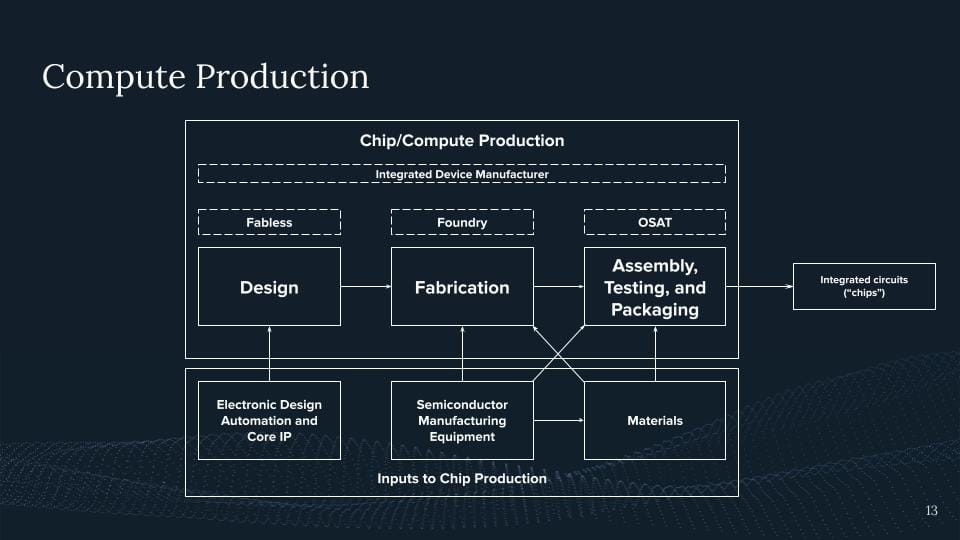
So one way to highlight this is if you look at the compute production, the compute supply chain. And what we see here is roughly all the categories that are involved in producing a chip.
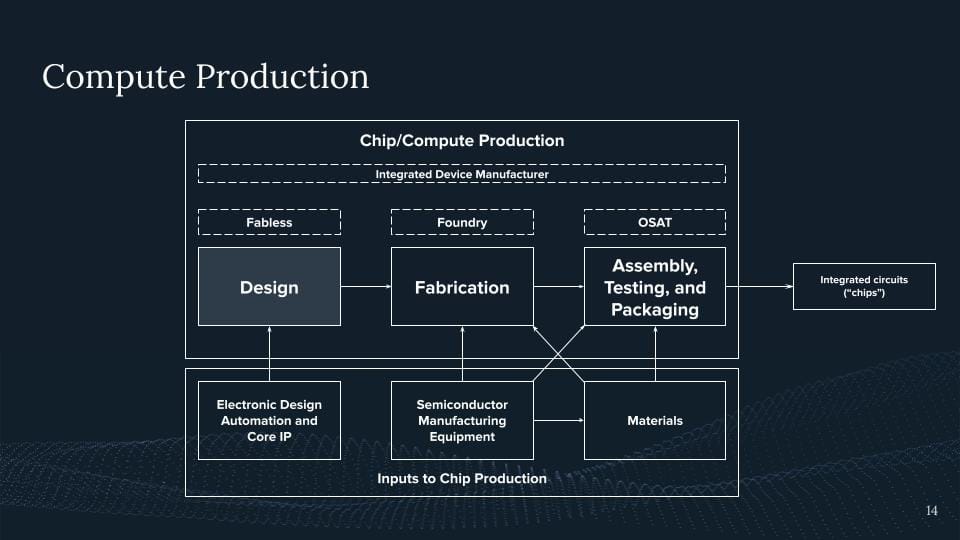
So where do we start? Where we start with the design. Somebody needs to design a chip. There are some designers here at Google for somebody designing TPUs or other chips. There are also designers from NVIDIA. And again, if we now take a look at this AI angle, it's just like, well, 90% of the market share of at least some GPUs you can buy on the market, if we now take a rendering out of it, are produced by Nvidia, right? 90% of these chips are being done there. We also have AMD, We have a bunch of startups in this domain, but right now we have a large concentration within Nvidia producing most of these design chips. And I think you've all seen it recently in the news with NVIDIA's recent revenues and how much, how big their market share, their valuation now is.
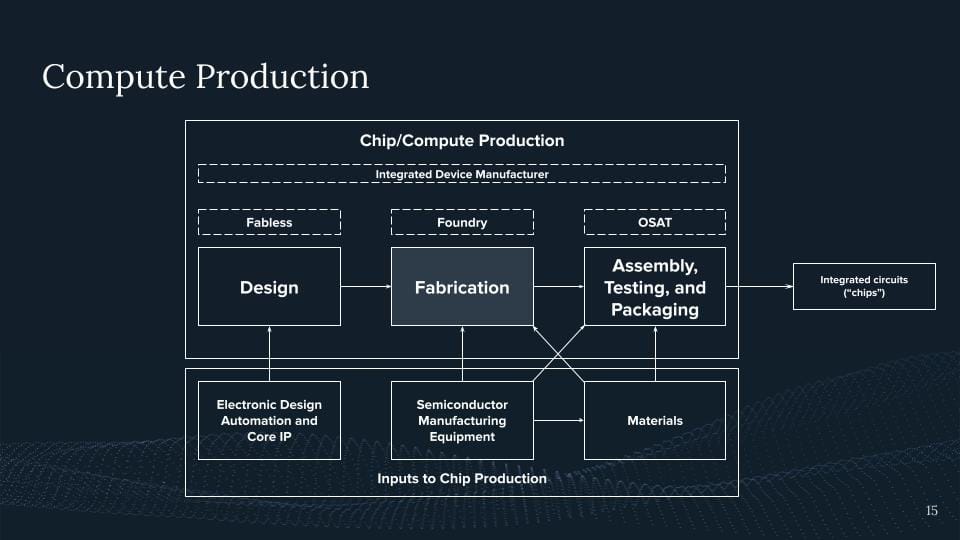
Think of it further, right? Somebody designs this thing, most Nvidia in this case does not produce it, they give it over to TSMC. They then have the machines that produce these chips. So again, if we look at the fabrication of these cutting-edge chips or anything below seven nanometers, this is limited to three companies in the world. This is TSMC, this is Samsung, and this is Intel with TSMC having the biggest market share for all of these chips. Again, TSMC, Taiwan, China, all of these things are like directly interconnected, right? But TSMC alone is not the only one, right? TSMC needs the machines that come from ASML because they all need to see UV machines, which we saw in the previous slide.
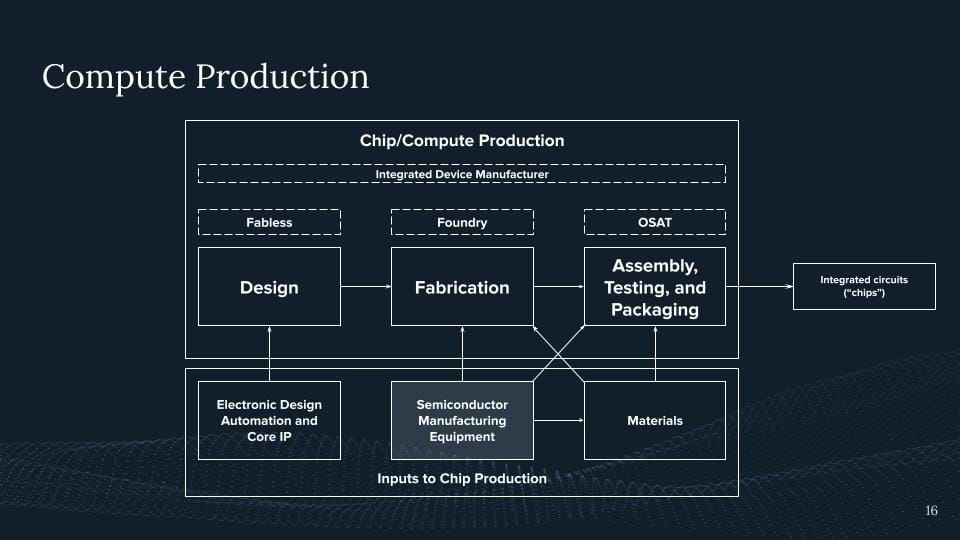
These UV machines are only produced by one company in the world sitting in the Netherlands called ASML. Again, 100% of these machines stem from there. But for these machines, you are not able to produce these cutting-edge
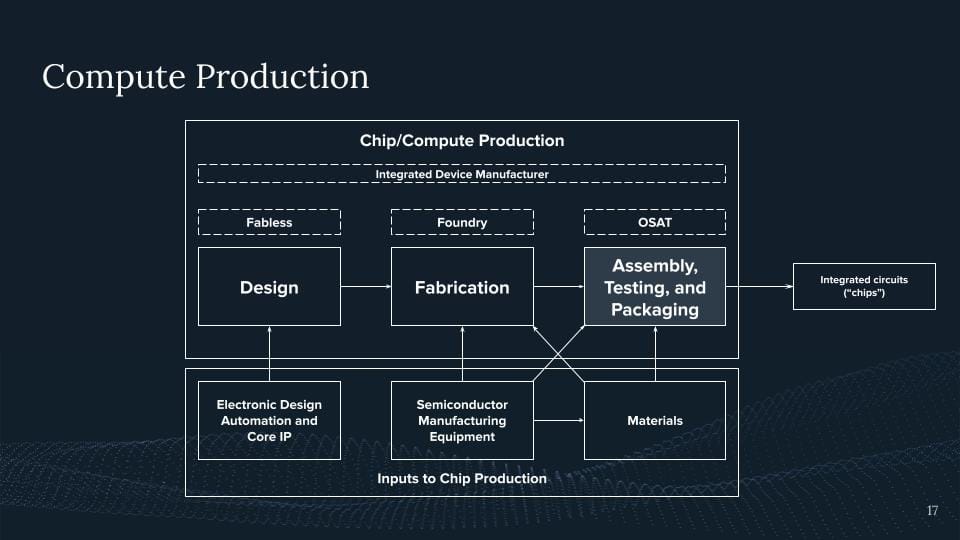
And then we go further, right? We have our chip, it's etched on this wafer, and then we want to do the same there. The reason why I'm highlighting this is here right now. This is part of the bottleneck we're currently facing if we look at NVIDIA H100.
These chips use something called HBM, high bandwidth memory. This high bandwidth memory is directly packaged with the chip to have the maximum memory bandwidth, eventually achieving this. And this is our current bottleneck. This is why we're not seeing more H100s out there and NVIDIA is trying to get more people to eventually do these special packaging techniques so you can package the memory and the logic together at the same time.
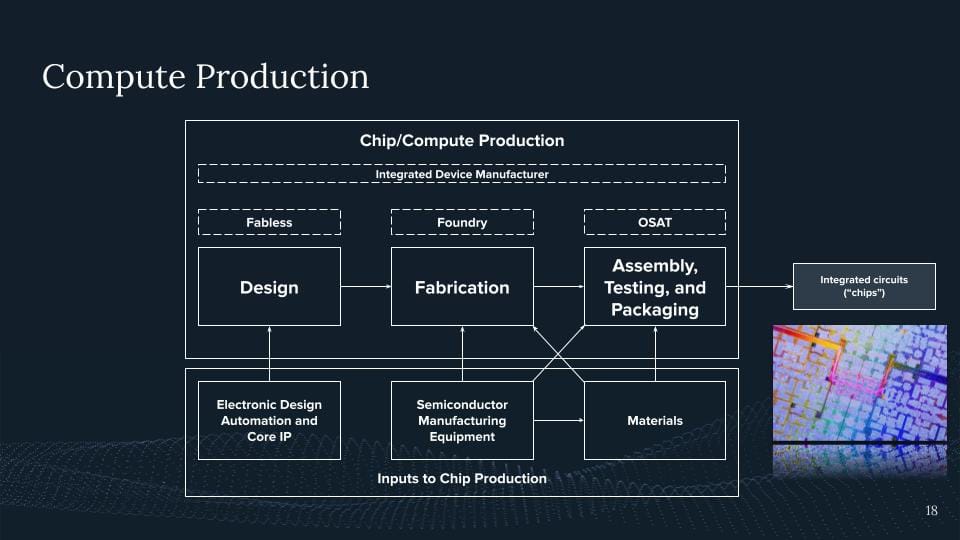
And there we go. It's our AI chip. Now we produce an AI chip and I'm claiming, yeah, that's a pretty complex product but hard for any one of you to produce this in the basement even for a whole nation trying to reproduce this. This is an effort basically of humanity on a whole national scale where a bunch of countries and a bunch of actors eventually come together and then we produce these products.
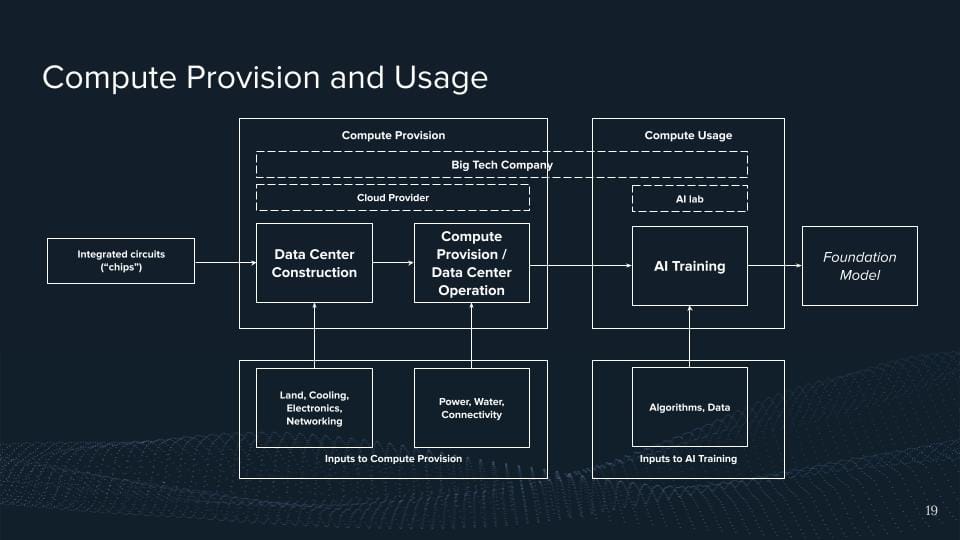
But there's no way it stops, right? There's a chip and if now, I don't know, depending on your job, if you're a software developer you probably have never attached any of these chips.
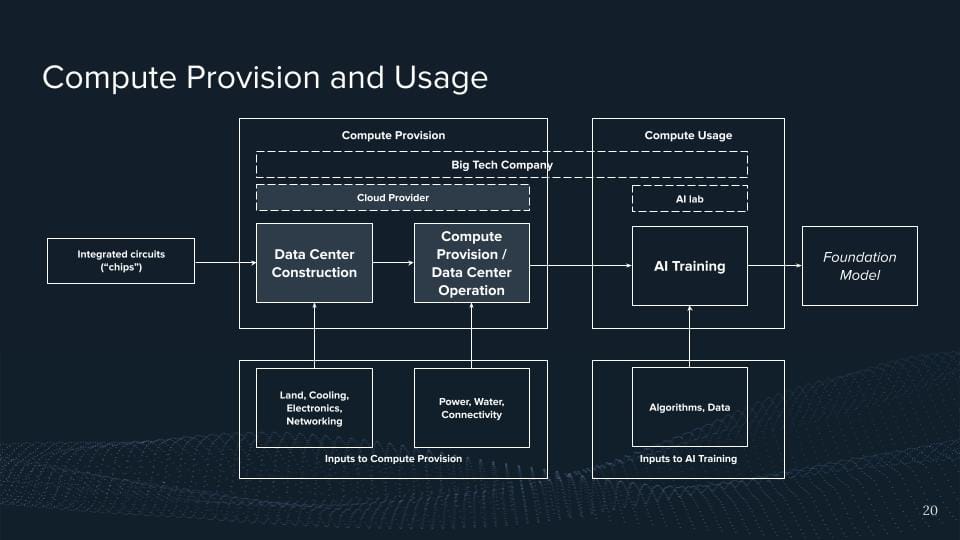
So this means these chips eventually go into these big facilities and the data centers which then help us to provide this. Oftentimes it's like simply saying the cloud, right? They run these data centers, then have a bunch of chips and they have like a nice interface to provide this. The reason why I'm highlighting this here again is that we have big market concentrations here. There are roughly three players that dominate in the Western market, that's Amazon Web Services, that's Microsoft Azure, and that's Google Cloud. And I would be claiming that the the concentration is probably even higher if we think about AI compute for these kinds of things.
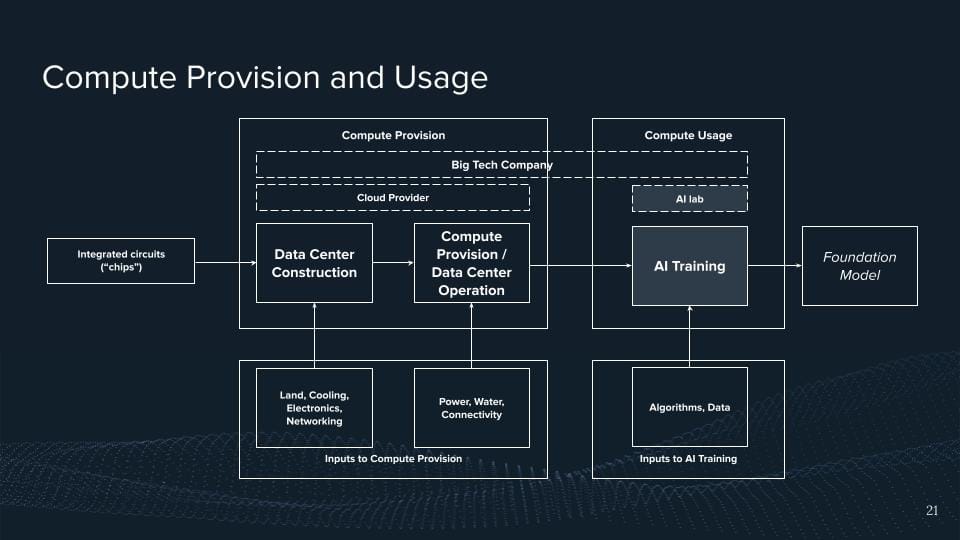
Well, in these computing powers, these chips are then eventually like being used by AI labs. And what we see is that a bunch of the leading AI labs have direct partnerships because of the importance of compute.
For example, OpenAI is a direct partnership with Microsoft Azure and for big S1 with Google Cloud. And if you don't have a bunch of people trying to have a partnership, I think hugging faces AI labs, yes.
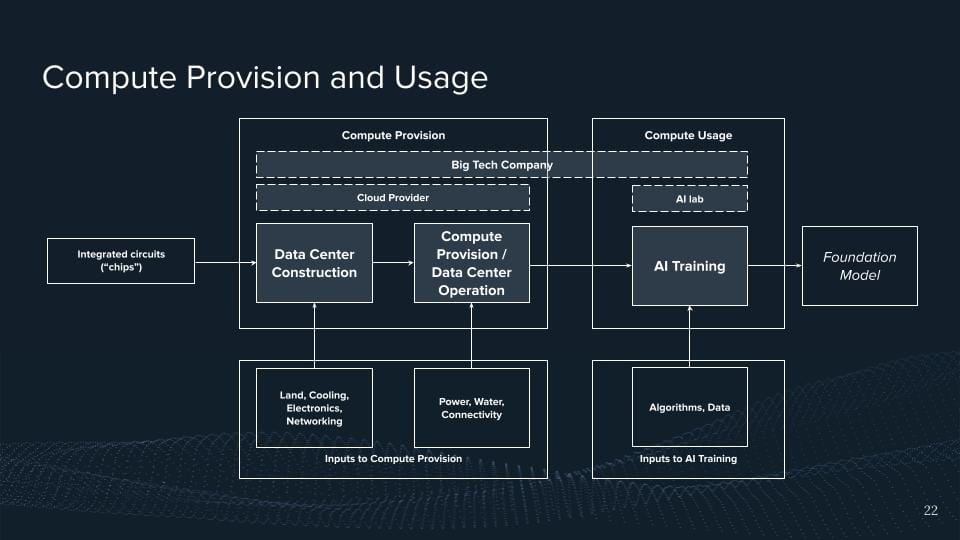
But sometimes it's just everything sits within the company, Google, and Microsoft being one example of this, right? You have an AI lab, and you're producing this AI stuff, but you're also running your cloud provider, and some of them are even producing their chips. So we'd like to see more of this integration across all supply chains, which is here highlighted just as a big tech company.
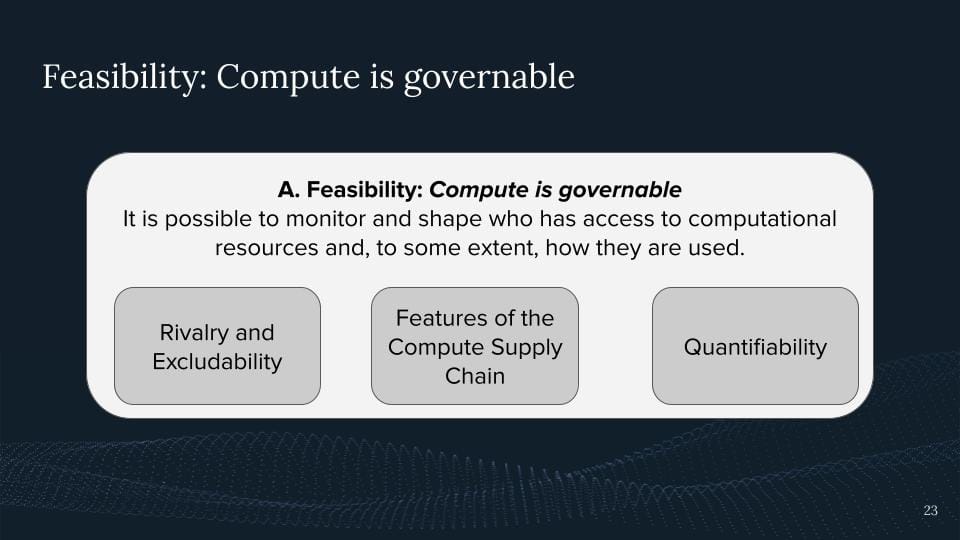
So going back to my previous original claims, like, well, here are some features of the supply chain, that I think make it particularly governable. I will later highlight an example of how these features of the supply chain already being leveraged right now. But all of these actions would be kind of futile, right? Like what I've just decided, like, well, it seems like you can count chips, seems like you just like kind of exclude people from it and you can have like, you have like some choke points across the supply chain. What's the matter for AI? Well, my claim is that computers are also indicative of AI performance, right? Now we come to the effectiveness part of this.
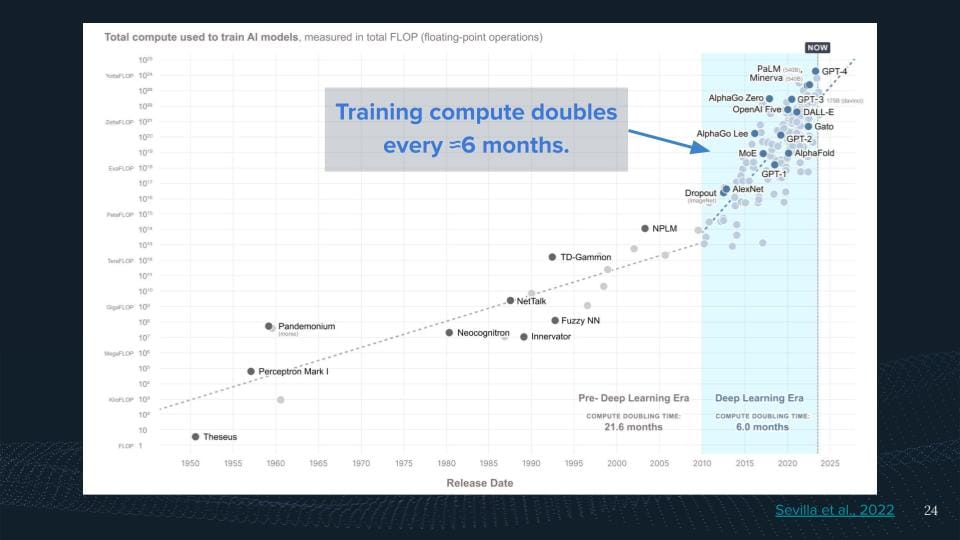
Why is this the case?
What we see here is the trend of compute being used for training runs of milestone AI systems. And what we see over time, this number went up a lot.
So we see with the emergence of the deep learning area, that the compute used for these kinds of systems has been doubling roughly every six months. This is actually how it all started for me. So we wrote a paper in the beginning of 2022 where we just like tried to estimate the computer usage for these systems and just learn what is the trend. Like how does the AI production look out? I try to scatter macro-machine learning research, just like understanding what's been going on. This led me to the conclusion, well, it seems like computers are a big deal. Maybe there are some nice governance properties that we can leverage there to have more beneficial AI outcomes.
Why are people doing this?
Well, if you double your compute use for these AI systems every six months, you spend more money. If you would just imagine you always spend the same money and just continue piggybacking on top of most long, you would double your computer every two years, because we roughly say chips get better. They double their performance every two years, and they double their price every two years. Whereas even there, it's like necessarily their price performance doesn't double every two years. What we see here is we double it every six months. This means we use more chips, and more money to train our AI systems. The reason why people are doing so is we get more capabilities out of it. You just see from more compute eddy systems, that they tend to perform better. Oftentimes the bitter lesson and the the most recently investigated in empirical research was scaling us, where we have scaling us for different types of domains. I'm particularly talking here about general-purpose systems or large language models, which are leading at the frontier here.
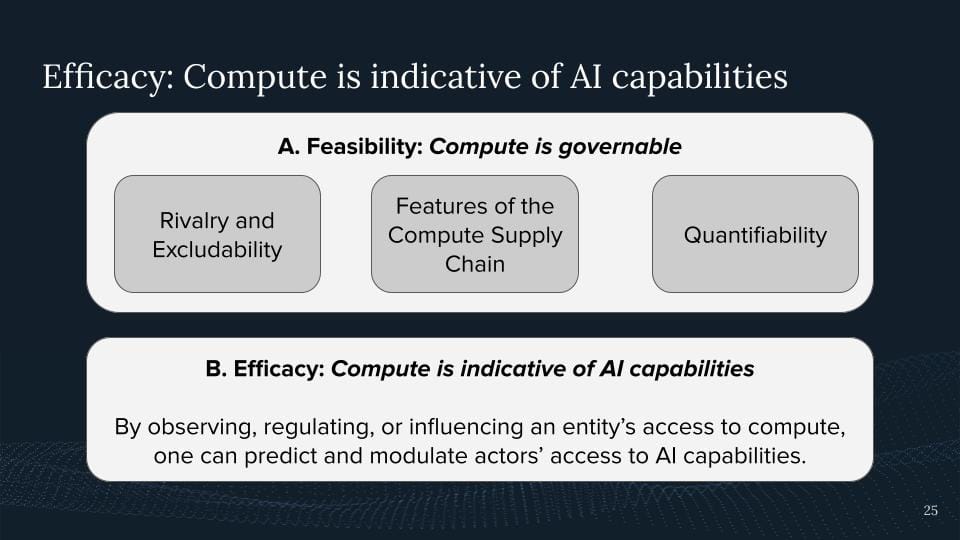
So it's feasible to govern compute, and I'm claiming it's also effective because compute is indicative of AI capabilities.
For example, by observing, regulating, and influencing an entity's access to compute, I can roughly predict and modulate an actor's access to AI ecosystems. I don't have a lot of AI systems in my basement because I just have my M2 chip on my MacBook which doesn't allow me to train any big systems, barely even deploy them. Whereas somebody is renting 20,000 H100s, they probably have way more access to AI capabilities.
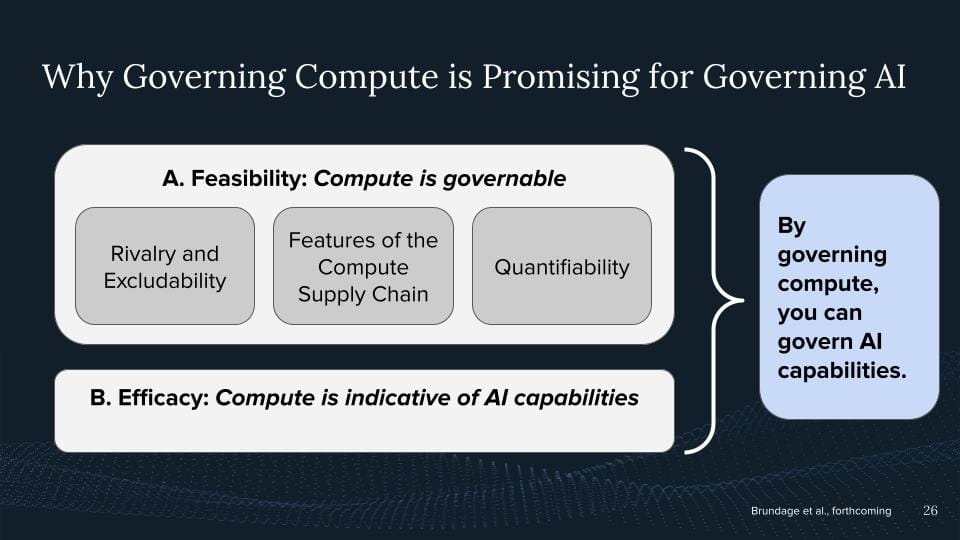
So putting this both together, I'm claiming, that by governing compute we can roughly govern AI capabilities, right? So this is to a large extent a theoretical and imperial claim you have been making, right? Why compute is such a nice level for AI Governance.
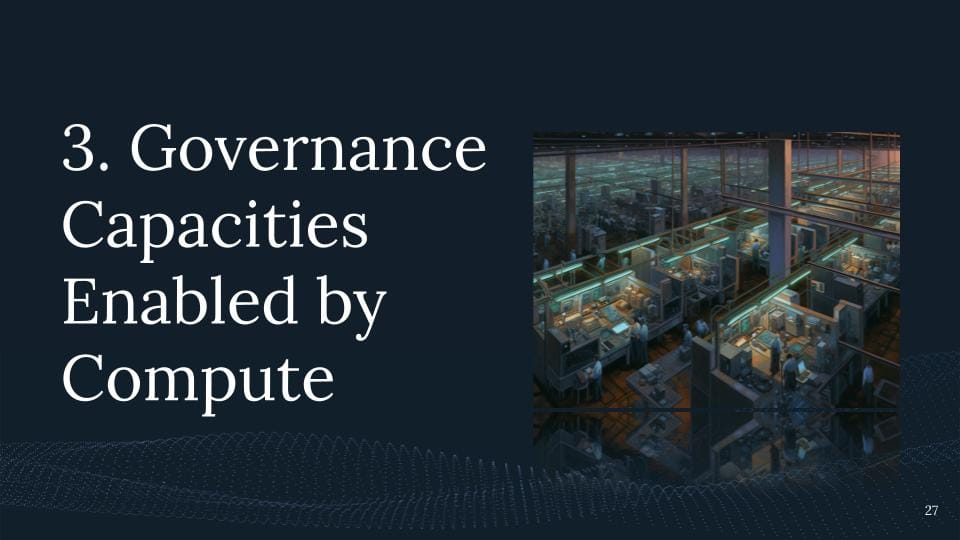
Let's go one step further, Well, it's like, well, what can we do now, given these properties? So now I'm going to be talking about the governance capacities enabled by Compute.
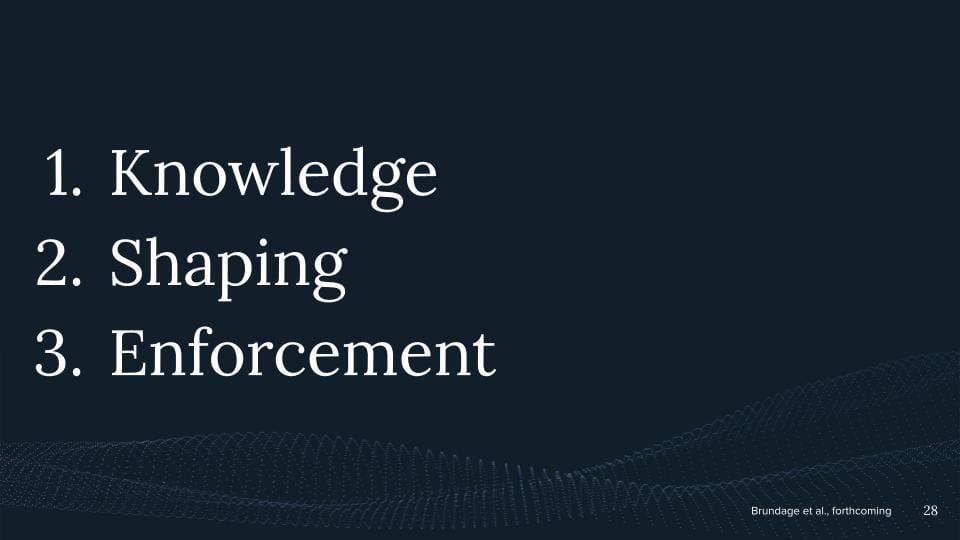
I put in roughly three groups, knowledge, shaping, and enforcement.
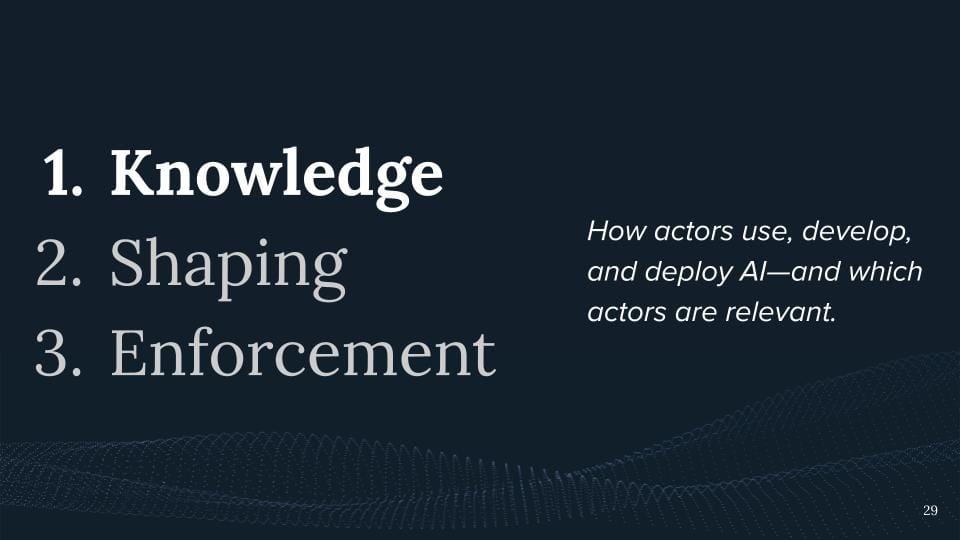
So the first one with knowledge, refers to the ability to understand how an actor uses, develops, and deploys AI, and which actors are relevant, right? This knowledge is crucial because it allows the government to make more accurate decisions and this way problems threaten the outcomes without other entities and just governments. So the idea is roughly, well, if you know who has access to sufficient compute, you know, like, you should care about it. This makes AI governance way easier. I don't need to care about every single being. I mostly need to care about people who have hundreds of millions in their pockets and access to these types of AI chips, because I only think they're capable of training these really powerful AI systems, particularly going forward into the future. And that's for example, the reason why I don't expect anyone of you to build GPT-5, at least alone, whereas I expect large corporations to be able to do these kinds of things.
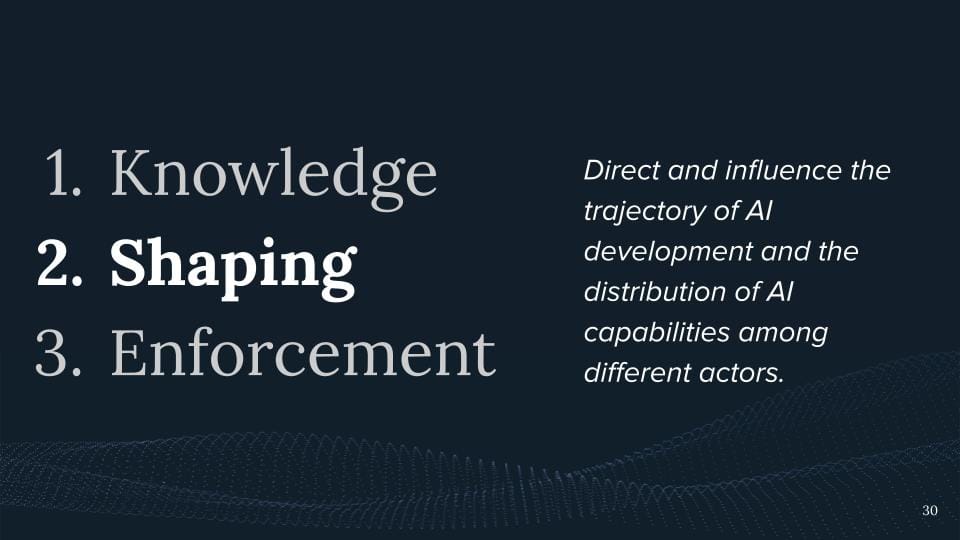
Well, a little bit further, a little bit more active than just passive is shaping. With shaping, we refer to the ability to directly influence the trajectory of AI development and distribution and distribute AI capabilities among different actors. For example, governments might want to correct market failures by nudging AI development towards more beneficial use in a way from less harmful use. We give the good guys more computing, we give the bad guys less computing. An example of this, before making our value segment, is the National Eye Research Resource in the US. We see the so-called compute divide. academics have less access to compute resources. This means they're not participating at the frontier of this research anymore, at least when we're talking about producing models. The National Eye Research Resource and the US are trying to address this, they're trying to give academics more access to compute, right? This is a good idea, and if everybody wants to do compute, and have research that's debatable, happy to share some more thoughts on this.
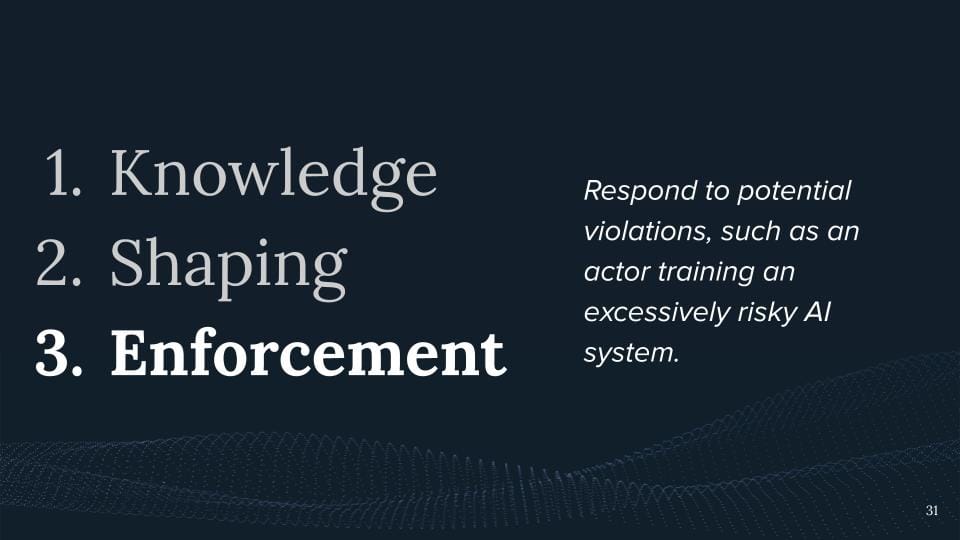
Well, we can also use compute as enforcement. So enforcement describes our ability to respond to potential violations, right? Such as an actor training risky AI systems. And we could achieve this via informal social norms, self-regulation, just law, and other procedures, right? The difference, the procedures will differ based on particular context. In this basic example, we can just take the computer away or make it hard for somebody with bad intentions to acquire sufficient computer training systems.
So again, to summarize, we know, roughly what's going on based on how much access they have to compute, then we have shaped by giving the good actors more compute the bad actors less compute, and lastly with enforcement which is a form of shaping right, we take the compute away from other actors but also like less more granular ways of doing this and like more less blunt ways of doing this which I'm more excited about.
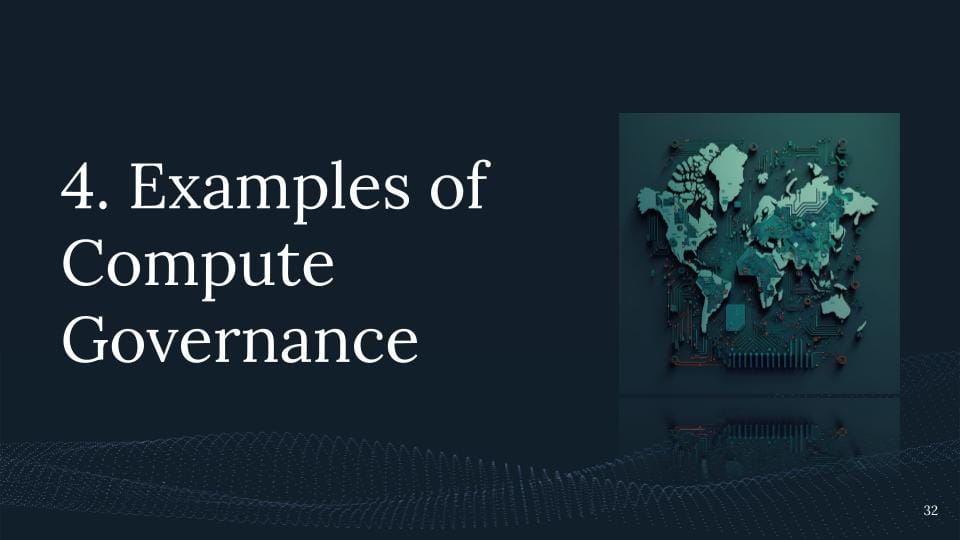
So what are now examples of compute governance? My claim is it's already happening people are currently trying to govern AI by using compute.
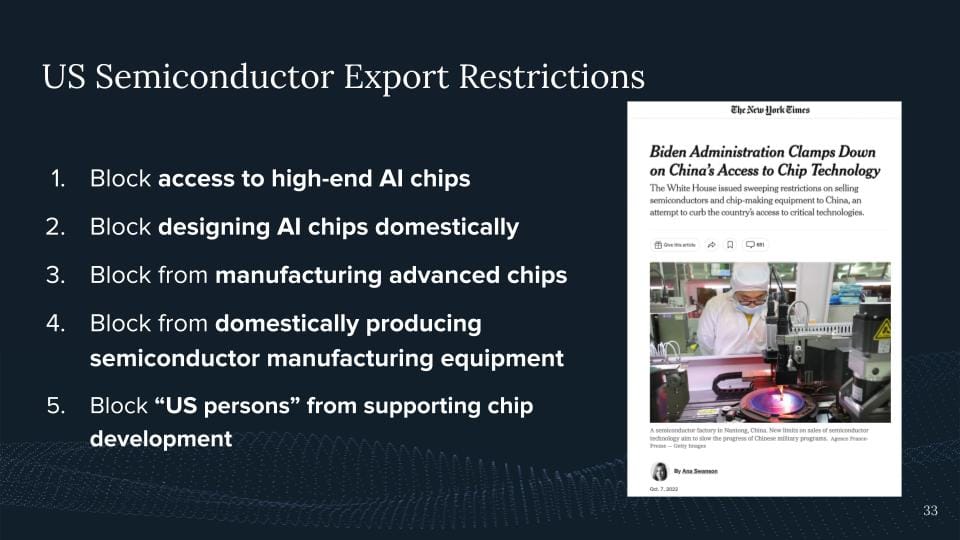
What is an example of this I think it's the US semiconductor export controls from October 7th.
So what did they do? Quickly run through it. They blocked access to certain high-end dynamic chips, right, the A100 and the H100 were not allowed to be sold to China anymore. That is, stop them from designing chips domestically by choking off access to design software from the US. Then they stopped them from manufacturing advanced chips by choking off them from the equipment you need to do this. For example, these ASM machines. Well, then they also choked them off from producing the machines by going one step further away.
It's like the input to these machines, SMEs, semiconductor manufacturing equipment. Well, I also stopped US people from supporting chip development. An interesting thing is that there is an explicit AI angle, right? It was explicitly listed by the US because of the rights violations of China and AI is becoming more of like strategic and geopolitical importance here and here it's just basically saying like well we don't give them the chips, we make sure they're able to produce these chips and that's a way of hindering the AI capabilities. If this is a good idea, if this is working, it's a completely different thing. All I'm just saying here it's happening people are thinking about compute as a tool for achieving this.
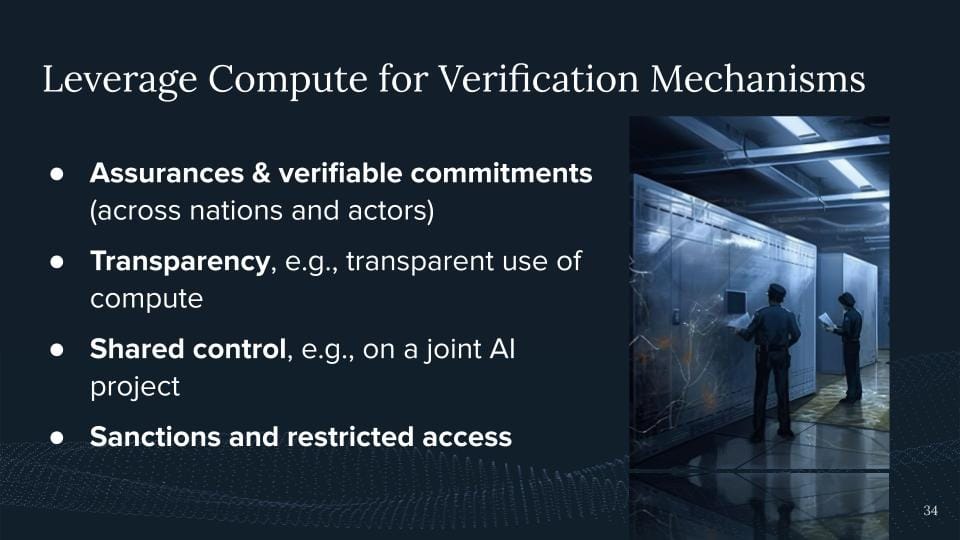
Another example is how we can leverage computers by building verification mechanisms around this. So I'm particularly excited about any type of insurance, very high-level commitments about AI capabilities, about AI inputs, about safety standards, about restraints. But also just providing transparency like how are you using compute? What is the largest training run you're doing?
And this allows us to dampen arms race dynamics if everybody would know right now maybe in a private space, how big the biggest training run is, people potentially like less be willing to race which I think would be pretty good because racing or just like moving even faster and as fast as any AI domain I think will mean we will be cutting corners and we will take safety less serious which I think would be a big mistake.
We might also want to enable some shared control on a joint AI project. We could just have a shared AI compute cluster where everybody gets an off button or everybody's able to see what the album is doing just at least roughly how much compute they're expanding on like what kind of task.
But we can also just like generally have just like sanctions and restrict access right for example by not giving people compute and by preventing them from a crime. Enough computing to build this.
So all of the ideas here are just for reducing your social costs. We have a technical problem and I'm excited about technical solutions which allow me me to say, "Hey look, this is what I'm doing. You don't need to trust my word, trust the math." That's why I call it Verifiable Mechanisms.
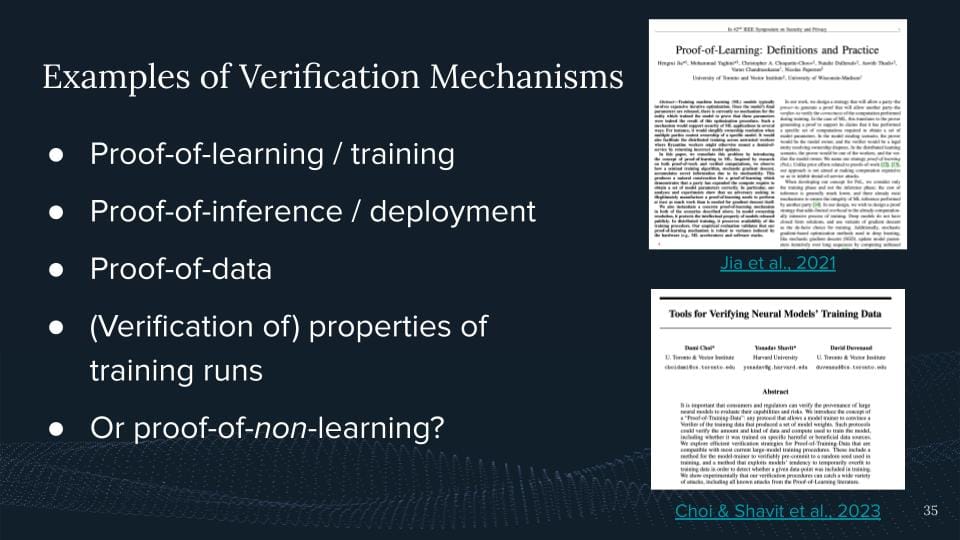
An example of this, which I listed here, highlights two papers, the proof of learning paper and the paper tools for verifying new neural models training data.
So the general notion is like, "I want to prove that I've learned a certain system. I want to prove I use that much computing to produce this system. If I didn't know how many chips you have, I can roughly know, oh, it looks like 50% of your computers here went to training the system. That's cool. I know you didn't train any bigger system. With this type of information, I can then make these claims, these verifiable claims with this proof of learning algorithm. But the problem is most of the compute, for example, is used for inference. So I also need to prove that I deployed the system. Not saying this paper doesn't exist yet as proof of inference, but I want some proof of deployment. I want people to claim, look, I deployed the system, I didn't train it to have a better idea. And also just prove that you deployed the system you're claiming to deploy.
We could imagine in the future, you're only allowed to deploy version 1.5 of safety mitigation acts, and then we just really make sure like, did you deploy the system we were talking about, or did you deploy like a previous version without the safety mitigations we agreed upon, for example, the government forced you to implement.
You can also have a proof of data. It's like, hey, you should not be able to use certain data for training. Copyright would be one example. What I'm more excited about is not training on certain harmful content, right? We don't probably want to train our model in 4chan. We probably also don't want to train our model on some chemical and biological weapons data.
And again, herring verifiable claims makes this way easier because I can just entrust them and trust empirical research on this and not particular people, which is particularly important if we think about like, states verifying each other's claims about AI.
I know if this gets me back to this idea of just like, maybe we want to have proof of non-learning, right? just claim like look I'm not training a system which is bigger than X I'm only training systems which are smaller than X I'm doing like climate modeling I'm doing all these kinds of other things which are not the thing which we agreed upon which we don't do.
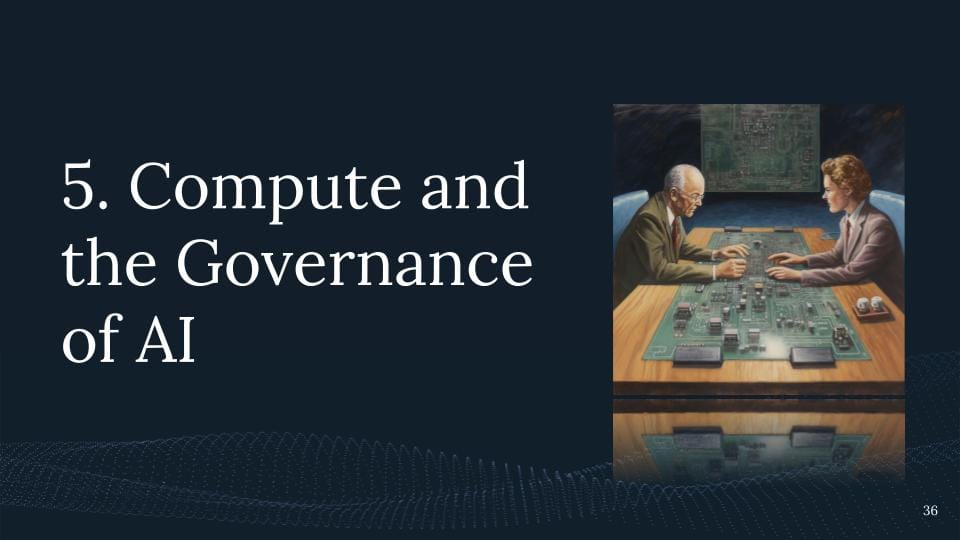
Okay coming to the closing now I want to take a step back again I like motivated compute as a node and as an instrument for your governance now just like when I asked the questions again. What are we trying to do with AI governance again?
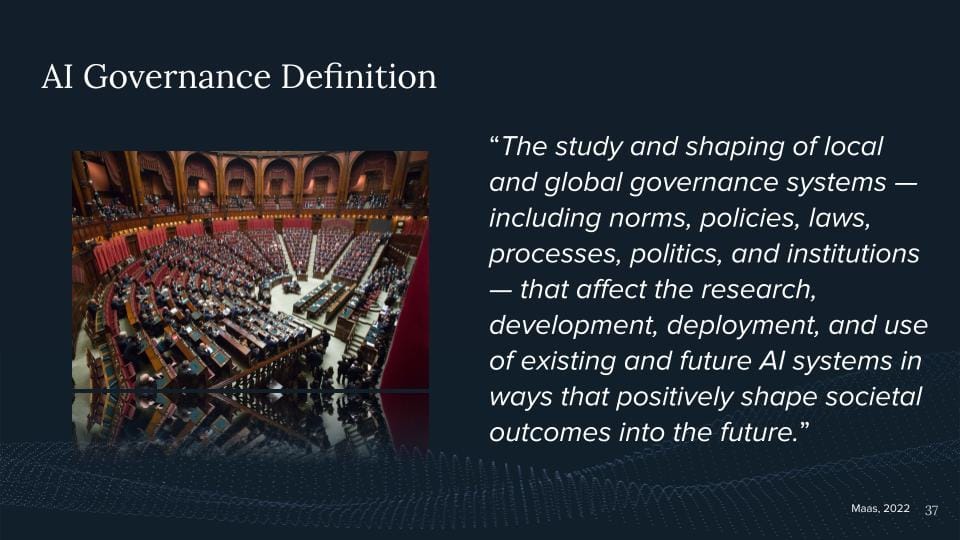
In the previous slide, AI governance is a bunch of things and I'm not providing the solution here, It would be mistaken to think, Oh, we're just going to do, govern a computer and we are good. I think it's an instrument. It's part of the solution. It enables you certain things, but it comes short in certain hours. So we need, again, we need institutions, we need other researchers who come together with this. And we had to send it for the guns off. Yeah, we tried to do so by having an interdisciplinary approach to this. And I just give my colleagues like, hey, look, here's a tool which makes it easier for you to trust people. That's the general notion of what I would go about. So what I want to do now is run through a hypothetical governance, policies that we might have in the future, and how compute can help there. I'm not providing all the solutions. I'm just like, look, here is compute being used as an instrument to provide some idea of what we can do there.
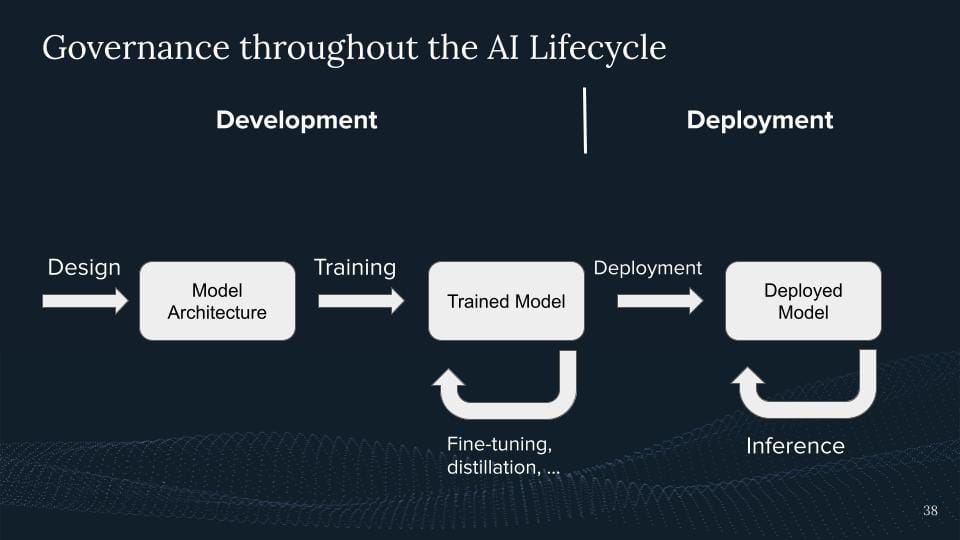
So let's quickly run through the AI governance cycle. We have a design. So we have model architecture. Then we train the system. Then we have a trained model. This trained model can be fine-tuned. Some kind of distillation can be going on. we can also do reinforcement from human feedback. A bunch of things you can do. And at some point, you're going to deploy this. And you have this deployed model in the world, which users aren't acting with. This thing gets continuous deployment of inference. So we can roughly say, oh, they're too weird. They're to things. There's the development. There's the deployment phase.
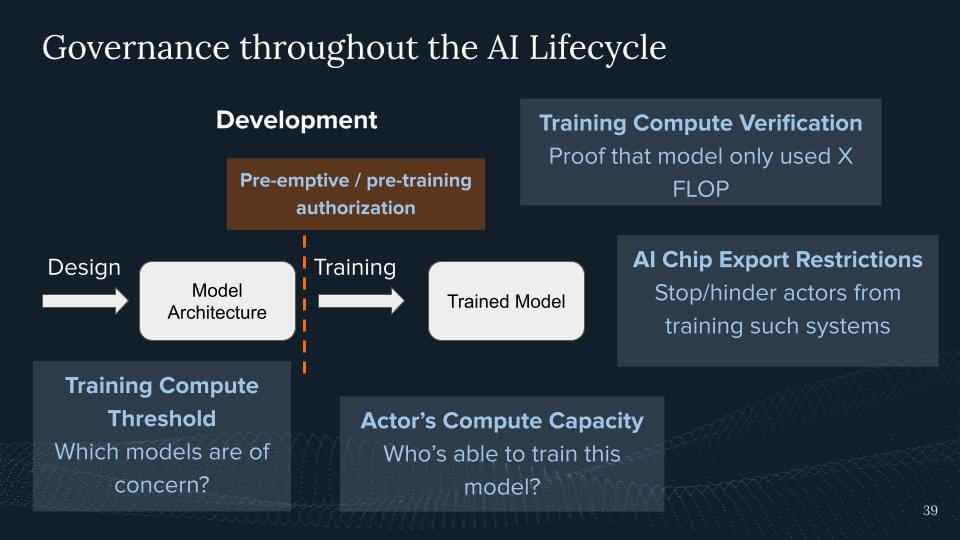
One thing we might want to do is a so-called preemptor for pre-training authorization. We want to say, well, you're only allowed to train a system if you fulfill certain criteria.
So a really good example. I cannot make any compute claims here because like neither can I evaluate this model because some models don't exist yet. So which kind of claims could I check? Well, I could, for example, check if you have a responsible AI developer.
Do you have certain risk management things implemented? If you want to run your bank, you need to fulfill certain criteria. I'm saying the same here. If you don't know what developer AI systems are, you need to fulfill certain criteria.
How could compute help here? Well, we don't want anybody who's training any AI system to fulfill it, right? not enforceable. So compute can help us like which systems are even of concern. It's like well we only talk about systems that use more than x-flop for being trained, right? Which models are of concern? I think that's a common way people now go about this.
In particular, we don't know yet the capabilities of the model, right? All we can say is like ah roughly this much compute and we can try to predict it. But compute might be able to give you a threshold and classify the systems you care about and the systems you do not care about, right? Because we but I want to reduce the regulatory burden.
We can also ask, who's enabled to carry systems? Who should we worry about? So if I only allow systems with modern X FLOP, then I roughly know, well, you need at least 10,000 A100s or something, and there are only many actors with 10,000 A100s, so there are only actors I need to worry about where I sometimes need to knock at the door and think, hey, how's it going? How is your responsibility, I develop this license?
Another way I can try to completely hinder this, is we just saw the typical export controls from the other side. It's like, well, I should stop actors from acquiring some computer resources from even training the systems. Again, those are all examples we can do there.
And later we can then also say, well, if they train a certain system, they should submit a proof of learning where they can show, look, it only contained that much compute, we did not suppress other thresholds because we want to have some continuous scaling, we don't want to go too far off the rails because then the model height might have more emerging and surprising capabilities. Again, all kinds of compute examples which help with this preemptive, training deployment. Again, not endorsing the solution necessarily, just highlighting this as an example of options.
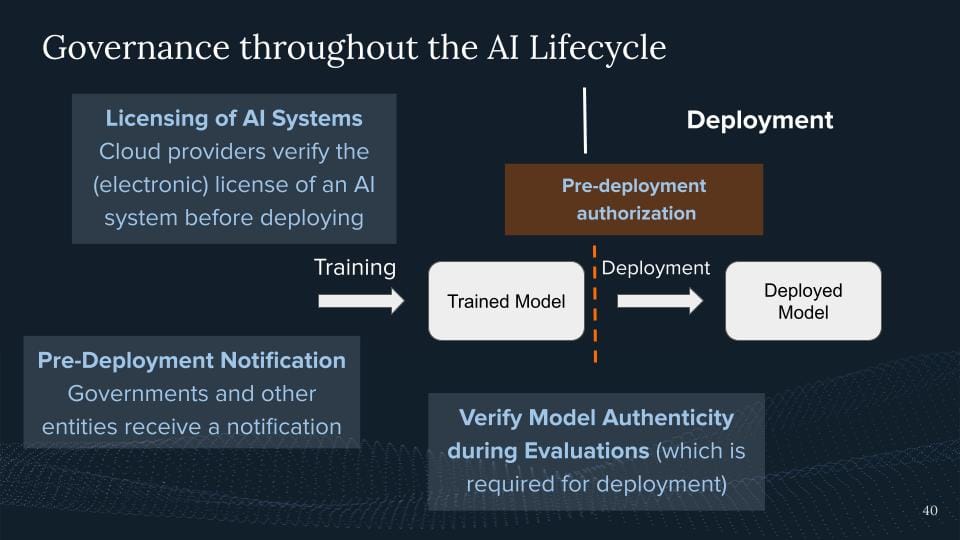
I think a more common thing, which we will potentially do in the future is, we're gonna regulate which AI systems get deployed. That's usually how we do governance, right? You can develop a bunch of stuff, what if you deploy something to the world, if you have users, you might be liable, and there might be certain requirements that come first, right? So we have like a pre-deployment authorization.
How could we do so? Well, we could be saying the models need to be evaluated. Again, I call up my friends who do model evaluations. So like good models, and bad models, they test these on various things, and they eventually give them the model certificate. And then the cloud provider, whoever the compute provider is, who then employs this model, right, because we create tons of computers to serve them or millions of users, they then just verify this license. This can be a bureaucratic procedure. We can also use electronic licenses to eventually do so.
Imagine your app store. Your app store from Apple or Google only runs apps that are signed by the app provider. Maybe we want to have something similar for AI models in the future. At least some system where people can check these kinds of claims if this model has passed a certain evaluation which we might want to check.
We might also just want to have a pre-deployment notification. We're like hey they give governments a head up that you've trained a certain system and that you might deploy this. And again cloud providers could be able to do this and again this could also be enabled like any system that was trained with modern x FLOP is doing this. But we can also make it like any system that is better at benchmark X, we should receive a deployment notification just for governments to get a heads-up.
And we also want to take some way to verify the model of Hentecity. So you evaluate the model, How can I make sure that the model you evaluated is also the one you deploy? Right? So there are also various mechanisms going back to verify the claims which are talked about how we might want to go about this.
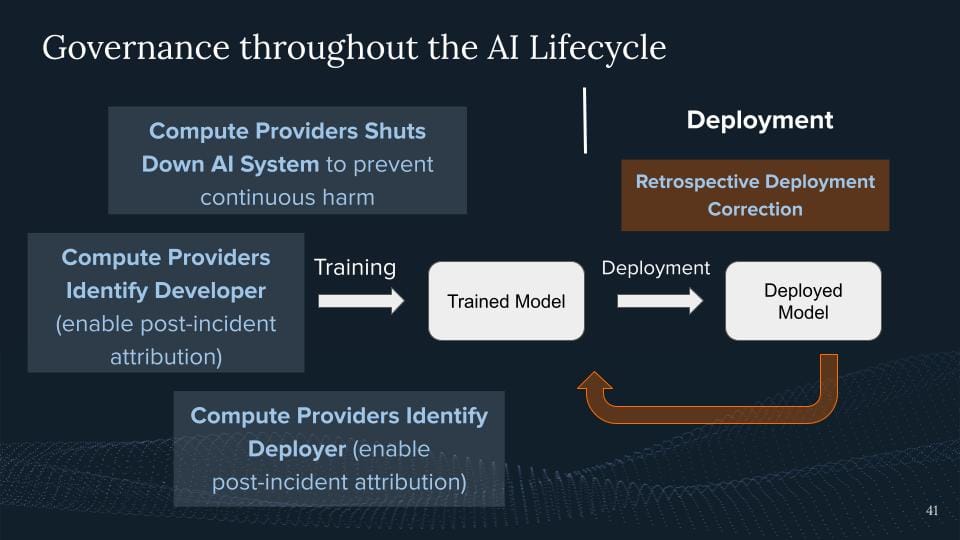
And then the last thing we might want to do in the future is a deployment correction because sometimes we deploy models, that are more capable than they are, or they go off the rails, and certain things happen that we are not excited about it.
One thing we can do is while the compute providers, they could help us identify the developer. Let's just imagine somebody is deploying a model, they run a massive misinformation campaign, and they're tampering with elections, so we just want to figure out who was deploying the system. To the same extent, if you would now go on the website and deploy a market where you would sell drugs. The police would call up your cloud provider and say, hey, who was this? And ideally, they had some know-your-customer regime in place. But it's one way to have some type of post-incident attribution.
They can also help identify the developer, which might be different from the deployer. It might be the case, Well, that some malicious actor deployed a Llama 2, and we just see this happening again, and we don't find these actors. We might want to have the developer liable for these kinds of things because they eventually enabled this, right? The employer would never be able to train a model like Llama because it costs millions, and requires a bunch of expertise, but somebody made it easy for them and this would be a way of going about it, right? Just by, for example having root access to the machines and just seeing like hey what kind of model is being deployed here? Or does this verify is like a licensed model?
An extreme case just like we just shut off the model, right? Because we also want the continuous harm from stuff happening, right? Again somebody runs this information campaign or any other malicious use we could imagine just clap over it just shut it down to the same degree It's like if I'm deploying a website where I do something malicious or which is not allowed we should just shut it down to stop the harm from happening right again.
This was like some examples where we can use computers or to across the life cycle and again we talked about like how can we have like authorization before you even train the system before you deploy a system and now lastly we talked about like what can you do to have like some deployment correction actually.
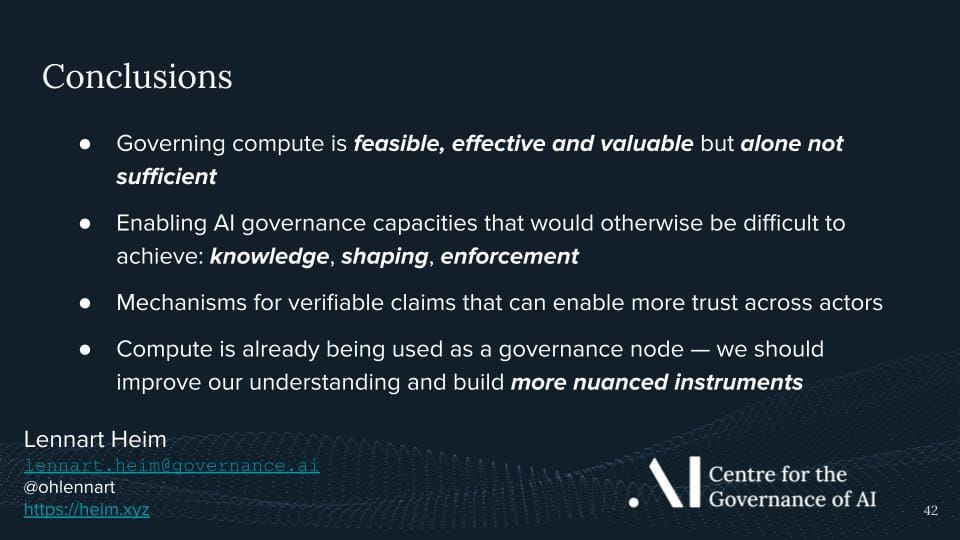
Okay, so putting all of this together. I've made the case that computers are feasible, effective, and valuable. We can do it and we're already doing it and it will likely have an impact on an actor's capabilities if you do something to that computer resource.
Again, it's not sufficient but it will have an impact that will increase the costs to a large extent and it gives us an instrument that is always really difficult to achieve because of these fundamental and unique properties of compute. But of course, this is not sufficient, right? This instrument should always always be used in conjunction with other policies. Again, we all need to come together and use it.
So I motivated a lot of us to gain the knowledge, right? Which actors are concerned, about what they could do? Then the shaping ability, like steering it towards more beneficial use of AI systems, steering it away from malicious use of AI. And it also allows us to be an enforcement tool, right? To just stop actors from developing and deploying these kinds of systems.
I mentioned that I'm particularly excited about these mechanisms for verifiable claims, right? Because they can enable us to have more trust in each other and reduce the social costs again across companies but also, particularly across nation-states.
And lastly, it's being used as motivated as the compute of the semiconductor export controls. It's already being used as an AI governance right now and I think we should try to improve our standing if our policy is chief or decide to go and maybe want to just have more nuanced instruments like this verifiable tool than just cutting off excess completely. Excited about these kinds of ideas, particularly going forward into the future.
Great, that's it for my side. You can find all of my contact details here. I'm generally happy to chat or reach out via email, Twitter, or whatever, just drop me a message.
If you have any of these things that you're like, "Yep, you want to work on this”, I've been keen, we need more people to work on these kinds of problems. I think all of you guys are like, particularly well-suited to work on these kinds of things.
That's it for my side and happy to answer some questions now.
Thank you.