AI Benefit Sharing Options
AI benefit sharing covers a spectrum of options, from participating in the semiconductor supply chain to accessing end products of AI research, with intermediate stages like providing structured access to AI models.
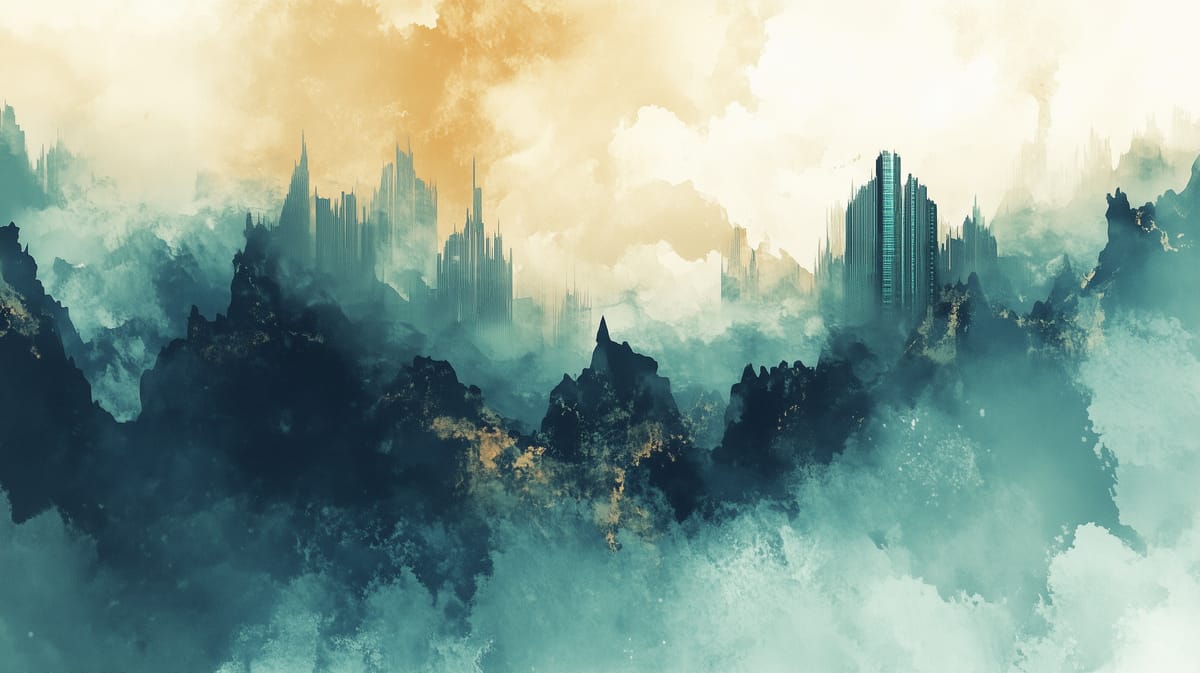
In discussions about AI benefit sharing, I think there are two critical themes that deserve more attention:
- Spectrum of Shareable Assets: What exactly should be shared? AI benefit sharing encompasses a wide range of elements—from input resources over sharing model access to final outputs and applications.
- Forward-Looking Perspective: As AI progresses, which future capabilities will be unlocked that make AI even more attractive? For example, pro bono AI worker sharing or cancer-curing drugs? The real importance of benefit sharing will become apparent when AI fulfills its economic promise, potentially having transformative impacts. It's in these scenarios of AI capabilities and profound economic impact that equitable benefit distribution becomes crucial.
This post explores the first theme by presenting a framework for understanding the options of AI benefit sharing.
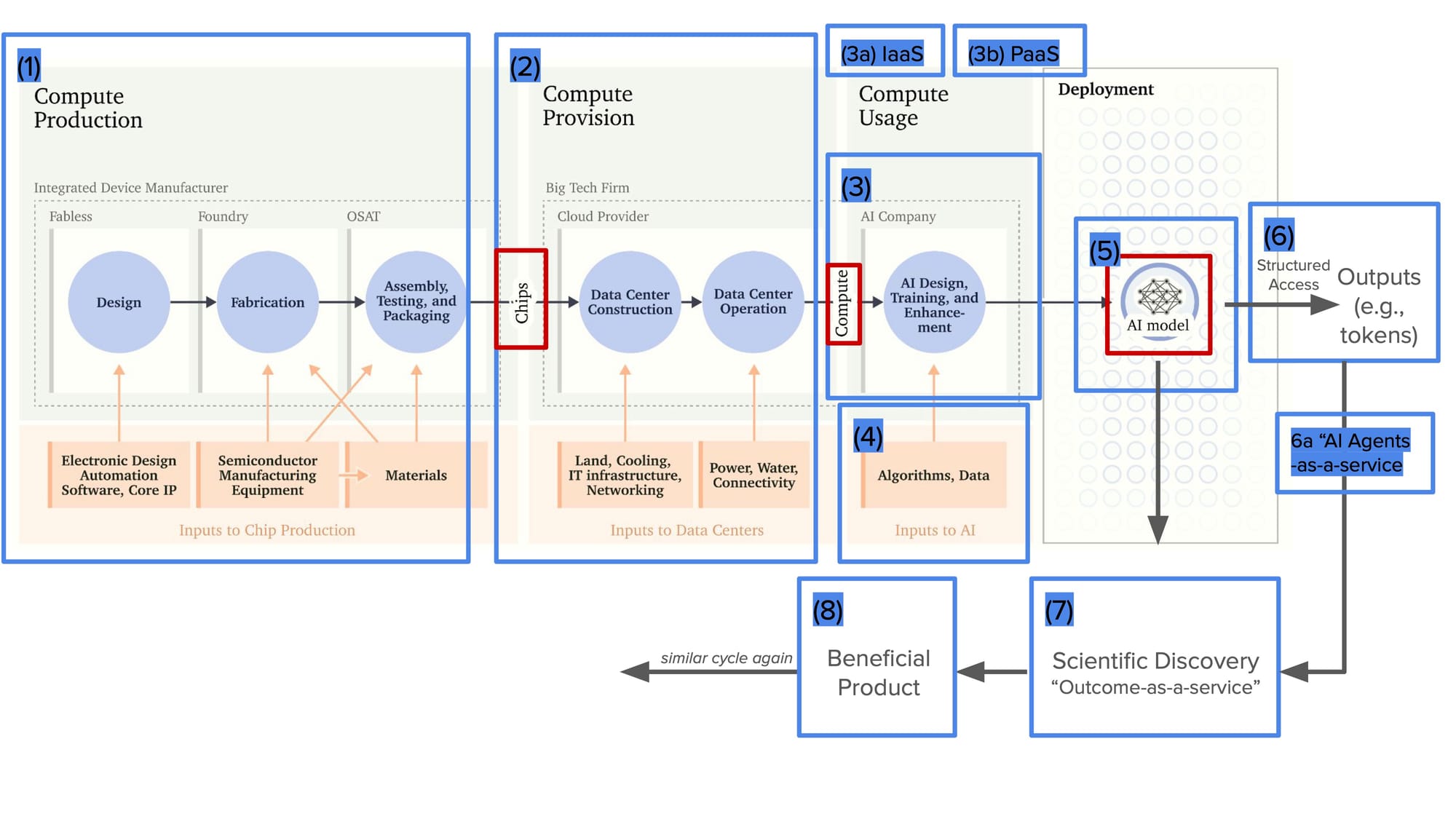
The figure above illustrates a view of the AI production and deployment chain, highlighting various points where benefits could potentially be shared. Let's examine each element:
- Semiconductor Supply Chain Participation: Sharing revenue from the AI economy by participating in chip production (and potentially producing your own AI chips).
- Advanced AI Chip Access: Enabling the construction of sovereign data centers.
- Compute-as-a-Service Access: Providing resources for AI training and deployment.
- AI Development Inputs: Sharing algorithms, data, and potentially talent.
- AI Model Weights: Granting access or sharing the core of trained AI models, the model weights.
- Structured AI Model Access: Offering structured access to AI models (e.g., via API).
- Scientific Discoveries: Sharing breakthroughs resulting from AI research (e.g., paper solving cancer).
- End Products: Distributing AI-enabled products or services (e.g., drug healing cancer, AI coding assistant).
For each element, we could consider two additional dimensions:
- Quantity: The scale of sharing (e.g., 1,000 chips vs. 100,000 chips); which can significantly impact the national security part of this discussion.
- Quality: The level of technology shared (e.g., access to H100 vs. V100 GPUs, or sharing GPT-4 vs. a hypothetical future GPT-6) affects the value and impact of sharing.
Discussion
The fundamental point is access at early levels of the chain does not guarantee access at later levels of the chain, since you won't necessarily be able to use the resource competitively. For example:
- Supply Chain Participation: Early involvement in the AI supply chain, particularly in semiconductor production, theoretically enables downstream options. However, participation doesn't guarantee competitiveness. For instance, while a country might produce AI chips, it may struggle to develop world-class AI models.
- Infrastructure Access: Access to advanced data centers provides (2 or 3) the foundation for AI training and deployment. Yet, having the infrastructure doesn't ensure the capacity to participate at the frontier. Some entities might find greater value in structured access to frontier models (6) rather than developing potentially inferior sovereign solutions.
- Model Access vs. End Product: In some cases, direct access to AI models might be less beneficial than access to the discoveries or products resulting from these models. This is particularly true for entities lacking the comprehensive infrastructure or expertise to fully leverage raw model access.